Being Human in the Age of AI: How to Responsibly Introduce AI into Healthcare
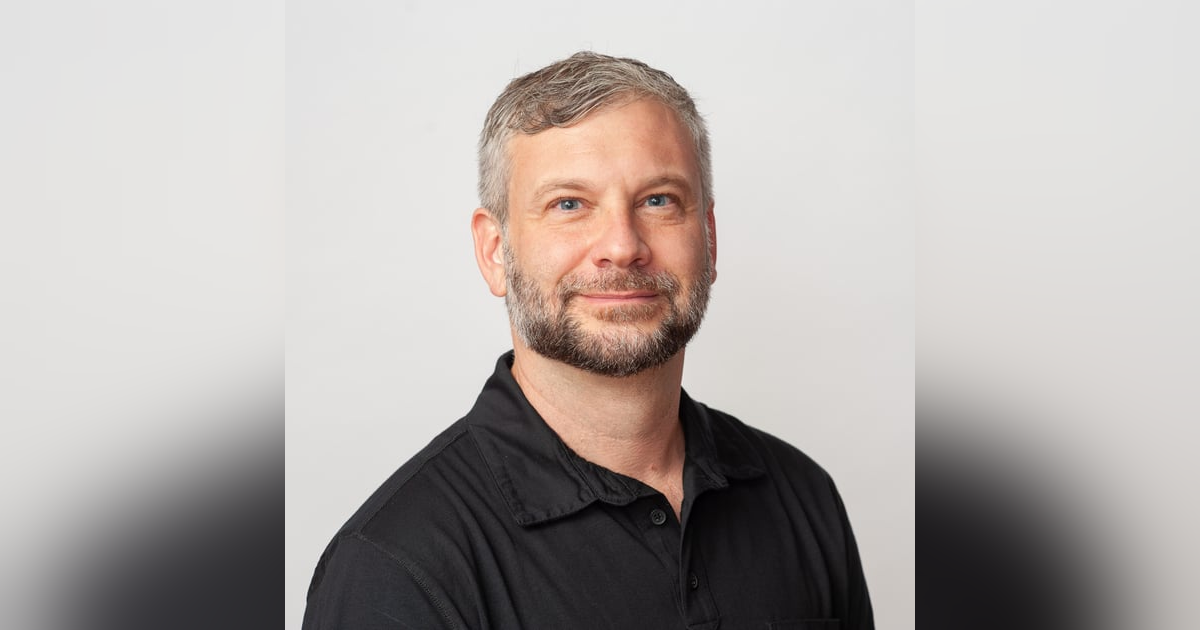
Dr. Greg Corrado, Distinguished Scientist and Head of Health AI at Google Health, explains how to responsibly introduce AI into healthcare.
On Season 4 of the Theory and Practice podcast, hosts Anthony Philippakis and Alex Wiltschko explore the many aspects of what it means to be human in the new era of artificial intelligence: from communication to robotic surgery and decision-making.
In episode 1, Dr. Greg Corrado, Distinguished Scientist and Head of Health AI at Google Health, explains how to responsibly introduce AI into healthcare. AI has proven itself in detecting diabetic eye disease, managing the risk of cardiovascular disease, and even encoding medical knowledge to answer patient queries, among many new and exciting applications.
Greg discusses safety concepts in AI: bias, robustness, transparency, explainability, and groundedness. He also discusses developing and maintaining datasets reflecting real-world patient realities and values.
Following this conversation, Anthony and Alex discuss Brian Christian’s book “The Most Human Human.”
Anthony 0:06
Welcome to GV Theory and Practice series four. I'm Anthony Philippakis.
Alex 0:10
And I'm Alex Wiltschko. I'm incredibly excited to start off series four.
Anthony 0:17
You know, my friend, I'm so excited to be doing this with you. And I have to say this feels like a really special moment in time. We're midway through 2023. Earlier this year, the world was taken by storm with the rise of chatbots and large language models, especially Chat GPT-4. Similarly, Apple has unveiled the Vision Pro, which seems like one of the more compelling products in the metaverse space. I just can't help but feel like this is a moment in time, where we're seeing the real rise of artificial intelligence, and not just machine learning
Alex 0:49
And Anthony, I think that's what our series this time is going to be about. What does it mean to be human in an era where capabilities that we thought were only available to humans, are now being given increasingly to machines?
Anthony 1:04
Exactly. I think this is the right theme for this series. You know, if you think about last series, we talked about what are the groundbreaking biological discoveries that will be talked about in the future. This season, really exploring what it means to be human in an era of human-like artificial intelligence?
Alex 1:23
Absolutely. I think this season, we're going to be focusing on exactly that topic on artificial intelligence and what it means for us. We'll be looking at how artificial intelligence helps us to communicate to see and actually even helps us smell in supporting the most human of functions, which is decision making. AI is starting to do all of these things, as well as us, and in some cases, better than us.
Anthony 1:46
How is computer science making this happen? Our computers replicating humans are forging a different path in the way that a plane flies, but it doesn't have to flap its wings or eat seeds the way a bird does.
Alex 1:58
And that's just it, we're going to spend eight episodes really digging deep, we're going to get beyond the hype. And in some areas, we're going to get beyond the fear or trepidation. To understand what the leaps actually are in AI and robotics and what they mean for particularly medicine and healthcare.
And rather than get caught up in some of the weirder, wackier and more worrying applications of AI, we're going to understand how the developments in machine learning actually came about and how they're enabling computers, and how they're enabling robots to take on human-like functions.
Anthony 2:39
And we'll work out whether developments in AI help us to understand ourselves, as humans better. We’ll ask questions like, how will human functioning artificial intelligence influence us? How will AI compete with us? How will AI enhance us? And how will we change AI? And how will it change us?
Alex 2:59
And of course true to our title of Theory and Practice, we will be casting a critical eye on how this technology is applied in practice in the field of healthcare.
Anthony 3:08
So today, we're going to find out what happens in reality when human-like AI and robotics are added to healthcare. And what does that tell us about the elements of safety that are needed? And since we are both such enthusiasts for AI, we're going to question ourselves throughout the series. And we're going to question our guests about the bigger picture of AI and robotics, in healthcare, with an emphasis on safety, ethics, and where all of this is going.
Alex 3:35
I'm incredibly excited to dig into this. This is the I think the most important thing that we can focus on as technologists, as healthcare practitioners, as researchers, as scientists, we're in a moment in, not just our society, but in our species where things are changing so rapidly. And now's the moment to figure out what's actually happening. And the only way to predict the future, I think, is to have a very clear view of the present.
Anthony 4:03
So who better to join us in this conversation than Dr. Greg Corrado, Distinguished Scientist at Google Research? Believe it or not, he's actually also our first recurring guest on Theory and Practice. Having joined us already in series one, Greg, welcome back. And thank you so much for joining us today.
Greg 4:20
It's a delight to be back.
Anthony 4:22
Alright, so, since we last met, you and your collaborators have been quite busy to say the least. You know, having published over 50 papers on the use of AI in healthcare, and covering a truly extraordinary array of topics, let me just list a few of them, detecting diabetic eye disease, cardiovascular disease risk management, encoding of medical knowledge to answer patient queries, and even working out gestational age and foetal malpresentation and using this in low resource settings. So these are all amazing examples of using applied AI in healthcare.
Let's take a step back before we dive into some of these topics. What were the key safety features you considered before starting to work on using deep learning in these applications?
Greg 5:05
That's a great question. I really believe that the, the fundamental necessity that we have when we try to bring AI to the world, is to take a human centred approach. And I think that when you're, you're thinking about what the technology is going to do, it's really to try to form a complement to meet human’s needs. And that's been our approach in trying to bring AI to healthcare. Healthcare is fundamentally a human human interaction. We want to build tools that assist, support and extend people's ability to provide care for each other. And I think that that underlying principle is part of how you begin to think through not just safety, but efficacy, and working really closely with the people who will be using it in practice.
Anthony 5:58
So you know, as we think, specifically the safety side of things, and that's gonna be a lot of our recurring theme today. One of the issues that comes to people's mind first, is that of bias, and we often go to that first when thinking about AI safety. Maybe you can say a little bit, because I know you've thought very deeply about this.
Greg 6:15
Yes, yes. So when we think about bias, the first line of defence in bias is having something that's fit to purpose. So all machine learning systems learn from data. And learning from data that is representative of the population that you're serving, and is also representative of the task that needs to be addressed, is where we sort of make our first stand. Now, I want to caveat that, or extend that by saying that what bias is, actually depends on what the intended use of the product or system is. So I don't believe that it's possible or tractable to say that an AI system has no bias for any possible application. But what you can do is you can look at a specific application, for example, breast cancer screening, using mammography and say, “All right, well, this AI system is focused on image interpretation. These are the elements that we need to care for, so that we address patient's needs equitably”, and measure as you go and understand that there will always be the opportunity to improve as you extend to new populations, as you extend to new tasks, you necessarily need to extend those datasets. And you need to expand the metrics that you use to evaluate bias.
Alex 7:46
So where does bias come from in these medical datasets? I mean, there's lots of different people that could benefit from these types of tools across all different walks of life. So I guess what kinds of biases creep into these datasets, and then what are the steps to mitigate them?
Greg 8:02
So the machine learning systems are fundamentally based on imitation, which means that whatever patterns occur in the training data, they can recapitulate those patterns. And so a big part of the bias that crops up in these systems comes from the bias in the data, where they were sourced from, and how patients were handled previously. And so that means that either who was treated, or how they were treated, in previous medical datasets, can inject bias into these systems. That's why it's so important to look carefully and understand your data. In, in many ways, the first step for any artificial intelligence or machine learning project is actually data science, and understanding what data you're using, and what properties those data have.
Alex 9:00
So tell me more about that. How do you get around, or at least identify this issue of bias? And then what are the steps to mitigate it in practical applications,
Greg 9:08
One of the first things to look at is comparing the population that you're tending to use the system with, and compare that to the population that you're training the system on. And it seems obvious that whenever possible, we should match these things perfectly. But in reality, the datasets that are used to build AI systems are always retrospective. They're always things that have happened in the past, and have been curated and chosen to be examples to learn from. And so a big part of how we both measure and address that is to look at what data were used look for gaps in those data, for example, a population or a condition that is not well represented in then expand that representation in future versions of the training data and that kind of working closely with the data themselves is a big part of how we guide systems. But there are also techniques that we can use, after the fact, to shape how systems work, and change the way in which they learn along the way. So
Anthony 10:17
One things that I think I've, I'm hearing it as an overtone, and I know you've thought about this issue, as well as it relates to AI safety is robustness. And a lot of your group has done work on trying to figure out, let's say, in preventive medicine, or screening, how to make sure that the technologies are robust and work in the field, maybe you can dive into that some using, let's say, you know, bowel cancer screening or cardiovascular diseases.
Greg 10:43
So fundamentally, the way that we're thinking about it in this context, is how well the systems responds, when given cases that are a little bit outside of the distribution that they have seen before, or sometimes very well outside the distribution of things that we've seen before. The AI systems of today, when they see things that are similar to examples that they've seen before, they tend to do quite well. But the worry is that they would behave in an unpredictable way, when things are out of the ordinary, one of the most useful tools here is actually the ongoing monitoring of systems and understanding how their predictions play out. And that's part of why when you build an AI system and put it in the world, it's not something that you then forget about and leave on its own. It's actually something that needs to be continuously monitored, and evaluated. Because as the world changes, as new things appear, we want to make sure that these systems are learning and adapting and serving new patients, new populations and new conditions, as well as they served the previous one. And this is quite an extension of, you know, what is sometimes called post market monitoring in the medical device field, where you really have to track how the system behaves, and evaluate whether it's behaving as expected, so that you can respond in a timely fashion.
Alex 12:18
We've talked about a couple of different concepts and safety. We've talked about bias. We've talked about robustness, what do the terms transparency, and explainability mean?
Greg 12:28
Let's start with what transparency and explainability should mean in this context. So transparency generally, is kind of the idea that you can peer inside a system and understand how it was built, how it works, in general terms, you know, the basics of what data were used, what the intended functionality of the devices, what its level of performance is, what are the mechanisms that are at play that actually make the system work? That's transparency. Explainability usually refers to the behaviour of the system, not in general, or how it was built in the factory, but how it is working on this individual case. So for example, in a radiological space, let's say that we have an x ray and an AI system spots something that it thinks is suspicious that it wants the human radiologists to to look at further having the human be able to then interrogate the system and understand well, wait a minute, what is suspicious here? Why are you flagging this case? Why do you think that this is something that we need to look at? And actually be able to understand what is being said and done by the AI system? That is the explainability part, why did you come to this conclusion or decision or classification of the data?
Alex 13:54
How important do you think transparency and explainability are for the safety of AI in healthcare?
Greg 14:00
What I said at the beginning about health care, fundamentally, being a human to human interaction, people caring for each other means that as you build these systems, you actually have to make them be a collaborator and a partner and a trusted tool that the caregiver understands. And that means understanding the capabilities. And it means understanding the limitations, right? Every tool has its limitations, has good intended uses, and ways in which it hasn't been tested, but maybe is ineffective. And we want to make sure that humans that are using these technologies have a very natural way of feeling comfortable with how they do their job, and what it feels like what it looks like for the tool to be behaving well versus for the tool to be not accomplishing the intended purpose. And that's why I think transparency around how the system was built, what the intended uses are that it's been evaluated for, and what good performance looks like what we should expect transparency around those things is critical.
Alex 15:16
Perfectly clear
Greg 15.18
The explainability piece where we're actually we want to understand why did this system give this particular answer for this particular case? That I think is something that doctors expect and they really need in order to be able to understand when to trust the system, how to trust the system, and how to collaborate, how to work together. But the kind of explainability that humans need, it's easy to get lost in details. A human doctor doesn't want to know, the details of thousands, millions or billions of numerical operations that lead to the decision, that is not an explanation at the right level. The kind of explanation that the human wants is the kind of explanation that you might receive from a human expert or colleague or assistant, where, for example, in radiology, you might say, “Oh, you flagged this case as suspicious. What do you think is suspicious?” And actually have a machine system that can identify it spatially, for example, on a medical image, and even drafted a description about what is unusual about this patch of the image? And then the human can kind of evaluate? Well, do you agree? Do you agree that this part of the image looks unusual? Do you agree that it looks unusual for the given description, and then that allows you to fold that information that's being provided to you by the AI system into your own broader decision making process, which includes so many factors that are well beyond the scope of any modern AI?
Alex 16:55
I guess what I'm getting the appreciation of is, there's a lot of considerations that need to be taken into account to deploy an AI system and an especially sensitive area. So just kind of going over what we've talked about. And then there's one final concept I want you to touch on. So we talked about bias in the data machines learn from patterns, and they replicate those patterns, you have to be careful. We've talked about robustness, how does the system perform over time and the monitoring that's required to make sure that it's still doing what we want and actually getting better. And you've touched on explainability, and transparency, which are factors that are really important, because these are tools that work with people. The last concept I'd like to dig in with you on is groundedness. So tell us about your understanding of groundedness as this final concept and safety. And maybe you could give us an example of how you've used this in your applied AI projects.
Greg 17:45
So groundedness is fundamentally about trying to make sure that AI systems relate to things that we all understand in the real world, and that there's an ability to measure and evaluate whether there are errors that sort of seem to creep into a system, as opposed to hewing really closely to the facts of the case. And groundedness is something that has become an increasingly important matter for us to address as we've turned towards generative AI.
Alex 18:22
And by that, I think you mean instead of the systems we've been used to over the past 10 years that can extract patterns from images and sounds and make predictions about what's in those patterns. These are new systems that can actually generate sound, they can generate images, they can generate videos.
Greg 18:37
Yes, absolutely. It's about that pattern recognition, versus pattern production and continuation. And pattern recognition is a surprisingly powerful and useful tool in diagnosis, in care management, in prognosis and in understanding risk. But the most impactful pattern that we've seen these systems begin to generate is human language. And all of a sudden, we've given AI systems, the gift of gab. For example, to be able to help draft a medical note. These are the kinds of things that we think are going to be coming to the future of AI in healthcare, but poses new, fundamental challenges. And we really want to make sure that systems that are helping to compose things are not injecting noise or new ideas, things that aren't part of what's going on in the real world. And then when you see something that deviates from that, how do you come in and correct that and make it less likely for similar things to happen? And there may be use cases for which we can say the tool is not sufficiently grounded when used this way, right. For example, I don't think that we're at a point right now where you can use some of these generative AI tools to draft a medical note and, and imagine that everything that's in there is actually going to be factual. But by the process of monitoring groundedness and pushing for things to be more and more robust in that sense, that's how we'll get to that place. And I think that the techniques to get there harken back to explainability, which is that if you see something in a system, you want to be able to say, “Well, wait a minute, why did you say that? Show me the references”, be able to link that directly to the evidence that led to the synthesis or the drawing of that conclusion?
Alex 20:35
And does that feature, that's a really important one. Is that feature of asking an AI system to basically cite its work to point at where it got a particular piece of information from? Does that get added into the algorithm? Or is that something that gets tacked on as a separate system, which maybe uses explainability? Or transparency of an algorithm to go into what it's learned and pull it out? How do you think about building this?
Greg 21:01
In some sense, Alex, you've just laid out what I think of as the spectrum of possibilities. And it's the same spectrum that we use for, for explainability that we use there for groundedness. Which is that, do you bake it into the system? That part of what it does from the very beginning is not only draw inferences, but cite its work and show the reasoning as it goes just constitutively, versus a system where well, what you do is you actually optimise it for getting to the right answer. And then there is a post hoc or separate system, that is in some sense, an auditor, that's there to come to, to take that judgement, and then try to analyse how that conclusion was reached, and what the evidence is for it. And I have to say that I think that at the current moment, there's a lot of value, and I think, a certain sense of security, that comes from having the explanation system be a little bit separate. Where in some sense, it's an analysis of the output is part of how explanation works. I think that that's comforting. And it's comforting to us in the current era. But ultimately, I believe that the direction of travel that we're seeing in generative AI and large language models is one where conversational interfaces are going to be so useful and so powerful, that I expect that they'll become dominant. And that, for example, if you imagine interacting with an AI system that's reading chest X rays, you're going to receive its findings about you know, these are the things that are suspicious. And then you expect to be able to, in some sense, have a conversation, where you ask follow up questions, and you expect good follow up answers. And part of the reason why I think we're going to go in that direction is that that's the natural way that humans want to work. And that's the most flexible thing, right? Imagine that if you, if you have a separate system that's focused on explainability or grounding? What if the kind of explanation or the kind of grounding that you need in this particular situation is a little bit different than what has been contemplated before? The thing that's great about these conversational interactions is that these systems adapt very well. And you can ask them for a kind of explanation, or kind of evidence that maybe they've never been asked for before. And they'll do their best and that you as the user can decide, is that answer satisfying. Does it make sense to me? Do I see the evidence itself? And building that trust, I think, is the way that we bring these systems into having real impact. One of my colleagues, Karen DeSalvo has this, this saying that healthcare moves at the speed of trust. And it is an incredibly powerful observation when you really incorporate it. You have to believe and really understand that this is a team where the patient trusts the doctor and the doctor trusts their tools. That's really essential.
Alex 24:24
Well, let's, let's go even further in that line of discussion and really dig into the practice part of theory and practice. So what was the most important thing that you've learned introducing AI into clinical workflows? We've talked about some principles and some values for how to do this well, but practically across the various applied projects that you've been referring to, what's the, what's the most important learning that you've taken out?
Greg 24:47
There- I'm going to give you two so one that I was most heartened by in bringing these tools into the real world is that when people can really hold technology in their hand, and see that it helps, and that they themselves trust the results, and they understand, and they can interrogate, it's explainability. I think it just changes the conversation far more rapidly than I expected it to. And then I think that familiarity is a huge part of making these things more useful and more accepted, and more robust.
Now, one of the things that was most surprising to me was I didn't, I don't think I realised how typical and common and prevalent it is, for a practitioner or a physician, to feel comfortable that they should be able to use something outside of its narrowly defined intended use. So medications go through FDA trials for a very specific use of that medication. But doctors routinely prescribe medications quote, “off label”, which means that it's being applied to a patient population, like maybe no one in this age group was actually in the clinical trial, or it's being applied to a slightly different condition. An off label use of medications is actually an important part of the healthcare system. And when we imagined how AI would be used, I was actually really imagining that it would only be used for exactly the intended purpose that we had described. But in fact, as soon as you start doing real world monitoring, you see that there are other ways in which people are going to try to use the system and explore how does the tool behave when I push it outside of its comfort zone. And so I think that you have to be aware at the outset, that folks are going to do it. And that you either need to embrace that possibility and make sure that people are supported in exploring how the tool works in different contexts, even if it's outside of what you expected, or to have guardrails, that check and that understand how the system is being used, and to see whether it's being used in ways that are not the ones that you intended, or expected. But I think what was naive was that you could just state that this is how the system should be used. And then that you would get 100% adherence across the board that that would be how absolutely every doctor would use it.
Alex 27:29
It really is reassuring talking to you about this because we've covered some really helpful guardrails, and also some sensible, respectful approaches to implementation. I'd like to move us on to discuss more of the fundamental concerns that remain about the introduction of AI into healthcare. And I guess I'll start with the ethical principles of privacy and autonomy. So I've been reading a bit about home based smart care systems for home surveillance of people with dementia. And you know, just for the listeners with smart cameras in every room, and then an array of sensors in the room and on the person, you can get alerts set up to notify carers of aberrations. And from a care perspective you want this, you want to know if somebody is in an unsafe condition or needs some help, right? So what do you think the key issues are here in relation to ethics?
Greg 28:20
So privacy and autonomy are human values that we, we hold dear and have to be considered when you imagine how any system is going to work? Now, I think that what I want to convey is that privacy is fundamentally intention in some ways with representativeness and the ability to have data that we can learn as much from as possible. And I think that what we want to do is we want to approach every application and say what is the right balance of privacy versus utility? In this particular case, right. So, you know, for a home alert system, let's say, we do want some level of privacy, but the only way to get perfect privacy is to not have a home alert system at all, that would guarantee complete privacy. But I think that what you can do is you can identify the aspects of privacy that you're most interested in, that people really find most valuable, and optimise for those. So thinking about questions about where data are housed? What data are saved in the first place? Who has access to various data? These are the kinds of questions that I think, allow us to understand the variance of privacy, the parts of privacy, that are relevant, in this case for home monitoring, you know, it's not the case that, you know, if my mom has a fall, she would want it to contact me, I don't think that she wants a video of the fall, forwarded to half a dozen folks in a medical institution. So it's really about understanding what does the individual want? What's your level of privacy and autonomy? And how do we build systems that can accommodate that. And it may be that a lot of it has to do with individual choice, I don't think that there is, in some sense, one privacy configuration that we should all accept, I actually think that for each applications, we should have the ability to set privacy and to even set privacy dynamically, to be able to say, “Look, right now I just want to turn this off, I don't want to be bothered by this”.
Anthony 30:44
Now, I'd like to close with one final question, which is, let's project forward 10 years, you know, we're in this moment of 2023, when we saw the birth of large language models that have blown our minds, as we think about the role of AI in healthcare 10 years from now. So 2033, what are the biggest things that we should be worrying about and planning ahead for?
Greg 31:07
I think the things that I see in the future of how AI is going to operate in healthcare is that the current wave of technologies are going to, I think, finally demonstrate that if we want care that is available, equitable and distributed in a cost effective way, we're going to need AI to do it. And moreover, the dream of personalised medicine, of having care that is really tailored to your individual needs, and your individual values to extend the enjoyment of our lives in the way that we think is, is the right trade off…those things are only going to be possible when we have AI that is really broad based, but operates in the individual's best interest. I think the things that we have to watch out for and be concerned for are misunderstandings about what a system can and should do. I think we have to be very, very careful about folks who may oversell or aggrandize the capabilities of these technologies before they're ready. And this is something that happens historically, kind of repeatedly, that when there are technical breakthroughs, some folks may get ahead of themselves in terms of what these things are really capable of doing. And so I believe that open, methodical scientific measurement of what the technologies do, and sharing the ability to form them, to shape them, to regulate them, is how we're going to get there. So I think that it's about having the right kind of speedometer and braking system, to be able to say, we're moving at the speed in which we can actually address and incorporate change and feel comfortable with change. That's the way in my view, that you get to something that is both bold, but responsible. You govern the rate at which you change things. And you try to include as transparently as possible, as many people as possible and understanding what's going on. And that's how you build the bridge to the future that people are actually excited to walk across.
Alex 33:43
Greg, I think that this touches upon a quote that you said earlier, which is healthcare moves at the speed of trust. So, thank you so much for the fascinating and illuminating discussion. We talked on cautions, we talked on hope, we talked on how to do this respectfully. And there's a lot of food for thought for us to take into the rest of the series on being human in the age of human-like AI. Thanks for being on the show.
Greg 34:00
It was a pleasure.
Alex 34:02
Thanks, Greg.
Anthony 34:12
All right Alex, let's move on to the hammer and nails part of the podcast where you and I talked about a nail problem, or a hammer the solution in honour of our in person meetups in Boston many moons ago. Alex, what has this episode inspired you to think about?
Alex 34:29
Well, it's a nail. In fact, it's the core of our whole series here. And what I'm asking myself, when I'm looking at all of these new capabilities that we've given to computers that we've given to robots, we can play chess, with computers, we can play Go with computers, we can manipulate surgical instruments in surgery with robots, these are things that we used to only be able to do as people. But now we've given these abilities to machines. So what's behind all of it? Like, what's what's left? What does it mean to be human? When there's so many human-like capabilities that all of a sudden exist outside of us?
Anthony 35:07
You know, it reminds me of a book that I read a few years ago that I thought was really thoughtful by Brian Christian, named The Most Human Human, and it had a kind of clever premise for it. So you know, our listeners are probably familiar with the Turing test. And the basic idea is you put a human on one side and a computer on the other? And how would we ever know if a computer was conscious, it would be whether it could trick the human into making it believe that it was. And in some sense, that's the best we could ever do, in terms of proving that we've created artificial intelligence. And so every year, there's a competition where people put in their chatbots. And then they're randomised. And then there are a set of judges, and also a set of contestants. And the judges are randomised to either talk to a human or to talk to a computer. And they have to guess which one it is. And so at the end, they give two awards. One, which is the most human computer, which is to say the computer that was most often mistaken for human. And then they also give the most human human, which is the human who is most often least mistaken for a computer, I have to say, sometimes I really would love to see the results of the most computer human, myself. I wouldn’t mind wouldn't mind. I think I've known some people who could compete for that one. I have to be honest.
Alex 36:30
Yeah, maybe each of us could submit to that competition.
Anthony 36:32
Yeah. Yeah, actually, maybe, but maybe I don't want to know the results of my score on that one.
Alex 36.38
Yeah. Let's, let's keep that a secret, I suppose.
Anthony 36:40
Anyways, but the author kind of sets out on a quest to try and win the award is the most human human. And the book is a little bit of a meditation on what are the qualities that make us human, and kind of how amazing it is that this is a moving bar. And you know, at first, if you go back to Babbage, people thought, if you could make a machine that could do arithmetic, then you'd have artificial intelligence. And, and that obviously turned out to be not true. We got arithmetic electronically pretty early. And then people thought, Well, maybe it's the ability to play chess. And of course, once we had a computer that could play chess or go, still didn't feel like we had achieved real artificial intelligence. And so the book is kind of a meditation on what exactly are these core capabilities that would enable us to believe that we had created artificial intelligence. And it's interesting, this book was written well before, Chat GPT. But I can't help but think for many of us language would have been the ultimate test of humanity. And if you could make a computer that could converse and do language, that that would be true artificial intelligence. It would seem like we have that now. And yet, it's still murky as to whether or not we've actually achieved artificial intelligence.
Alex 38:02
There's this concept, which we've talked about on the show before. And it's an idea that I got from a colleague of mine, Hugo La Rochelle. And it's called the receding horizon of intelligence, which is, as we move forward, and as we do things that we've previously said, “Oh, if we could do this, we'd have artificial intelligence”, we find that the horizon recedes into the distance, that as we open up the systems that actually solve the problems that we've previously tacked on the wall as our goal. When you open up those systems, we find that we actually understand a lot of the parts because they were engineered, they were constructed, and we find that the mystery and the mystique actually leaves us in it. It retreats further on into the future that oh, okay, actually the bar is even higher. Because we now have the system, we can inspect it, we can see its flaws. And I think, doesn't seem like that process is going to end. But in the process, we have to be examining ourselves like, well, what what is it that's left? What is it that we want? You know that that to me is the question. I don't know if we're going to find an answer to this, but we're certainly going to ask the question over and over throughout the series.
Anthony 39:06
As we're talking, Alex, I find myself thinking more and more about what do I think it means to be human? You know, and again, one reaction right away, and this is covered in the human human book is about empathy. And yet, you know, there's an interesting paper we'll talk about later in this series, where it seemed at first blush like some of the chatbots might be showing more empathy than doctors. So kind of scratch that one off the list. Start thinking about some notion of having a mind's eye and the ability to construct a worldview. That seems compelling, but very hard to empirically falsify. So I'll be honest, I'm not sure that I yet have something that I find satisfying as the answer to this question. And as we go through this series, I'm really looking forward to delving into it more with you.
Alex 39:55
I completely agree, it seems the more we tug the thread, the more we find things we can actually understand and not some glowing core of great mystery, which is human consciousness, or what it is to be human. But it seems like as we unravel the sweater, as we pull the thread of our own humanity, we find more and more details, we find more and more to understand, more and more to look at. And we're very much in the journey right now. And if there is a destination, I feel like it's far off. But what I'm very excited to do for this season is talk to people who are in the journey as well, who are leading the journey in some cases. So I'm just very, very, very thrilled to be back here with you, Anthony in conversation, you and me, and also you and me and some truly, truly excellent people, as we explore what it is to be human on series four.
Anthony 40:50
I couldn't agree more, my friend. On that note, let's end today and revisit these questions over and over again.
Alex 41:00
Our thanks to Dr. Greg Corrado, for joining us this week. Next week, we'll be exploring how conversations with computers have become so human-like and what this means for healthcare. We'd love to know what you think. Write to us at theoryandpractice@gv.com or tweet @GVteam.
Anthony 41:17
This is a GV podcast and a Blanchard house production. Our science producer was Hilary Guite. And our executive producer is Duncan Barber. With music by DALO.
I'm Anthony Philippakis.
Alex 41:29
And I'm Alex Wiltschko.
Anthony 41:30
And this is Theory and Practice.