Mapping the World of Smell to Broaden Diagnostics in Healthcare
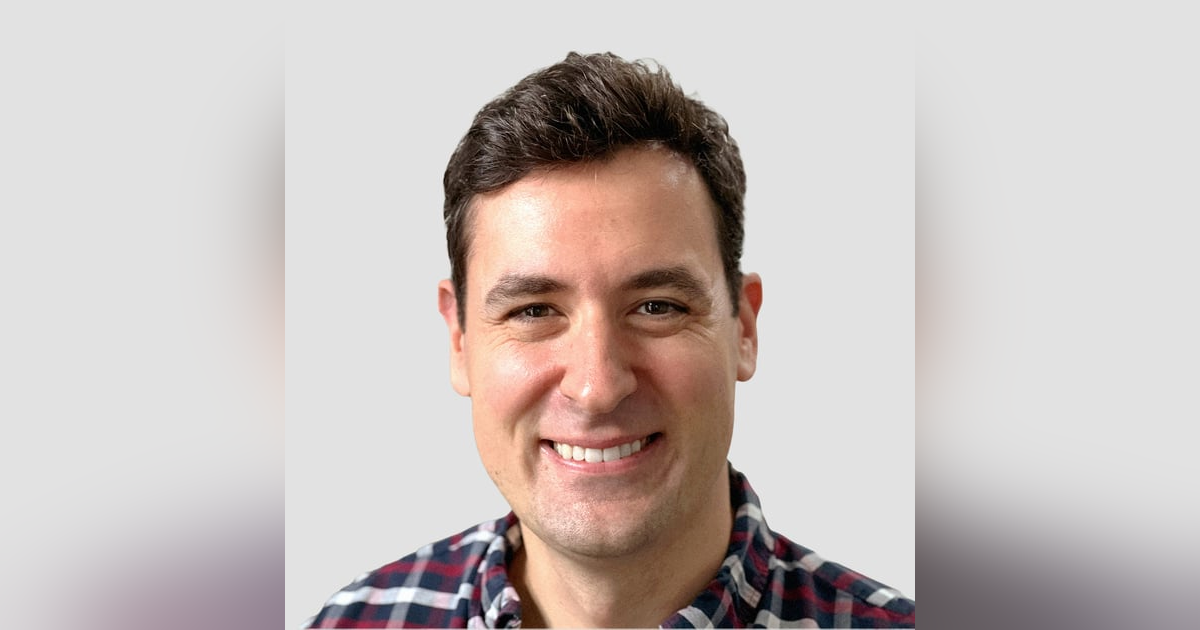
In episode 5, we explore how machine learning helped create a map of odor and how that technology will train computers to smell. Anthony Philippakis visits Dr. Alex Wiltschko’s lab at Osmo, where scientists are dedicated to digitizing our sense of smell.
On Season 4 of Theory and Practice, Anthony Philippakis and Alex Wiltschko explore newly emerging human-like artificial intelligence and robots — and how we can learn as much about ourselves, as humans, as we do about the machines we use. The series has delved into many aspects of AI, from safety guardrails to empathic communication to robotic surgery and how computers can make decisions.
In episode 5, we explore how machine learning helped create a map of odor and how that technology will train computers to smell. Anthony Philippakis visits Dr. Alex Wiltschko’s lab at Osmo, where scientists are dedicated to digitizing our sense of smell.
Anthony
00:01
Welcome to GV Theory and Practice. This series is exploring what it means to be human in the age of human-like AI. I'm Anthony Philippakis, on location in Boston.
Today I'm going to see my good friend and co-presenter of this podcast, Alex Wiltschko, who has recently set up a company called Osmo, which aims to give computers a sense of smell. Alex has always been motivated to understand the human sense of smell better.
He studied neuroscience at the University of Michigan because of its unique program, and then he chose to be a postgraduate at Harvard Medical School, simply because they had the most olfactory neuroscientists of anywhere in the world. In industry, Alex most recently worked at Google Brain, which was part of Google's AI division for nearly six years. There, he set up the Digital Olfaction Team, which has recently published the first digital Map of Odour. Last year though, Alex setup Osmo with funding from Lux and GV. It aims to enable computers to smell and maximize the impact they have on health and well being.
Anthony 01:03
It's a beautiful spring morning, and I'm just walking down Main Street here in Kendall Square from the Google office to go visit my good friend Alex at his new startup Osmo, where computers are being trained to smell. And I have to say, it's just kind of amazing that as we're walking into the building here, I have this incredible sense of smell of cookies being baked in the area. I don't know if there's a bakery, somewhere nearby, but it's like, I have this like sense of my childhood, and my mom baking cookies. So what a poetic and right way to start the episode. And, you know, and one thing that I find so amazing about being here in Kendall Square, is just how much incredible scientific activity is happening between MIT and then this world of startups that are changing biotechnology, so it's great to go visit Alex's work today. And we're just entering into the building here.
And oh, wow, this is so cool.
It's you know, such an incubator space. It feels just like any startup should feel. Young people everywhere, big open space, can see all the piping in the ceilings. What a great place to build a company. Hey, Alex, how you doing my friend?
Alex 02:15
Good to see you, Anthony.
Anthony 02:16
This is gonna be like a truly special episode, where this time you're the guest instead of the host.
Alex 02:21
It's a very strange role to be playing. But welcome to The Engine. Glad to have you here.
Anthony 02:25
Very awesome, my friend.
Alex 02:26
And the vibe here is really very sciency very deep, techy people are running around with like, one lab glove on people are moving, there's a lot of science talk, this is actually a really, really great place for Osmo to be - it's awesome.
Anthony 02:37
Should we go upstairs?
Alex 02:39
Let's do it.
The lift 02:42
First floor…. first floor
Anthony 02:43
Wow, this is very cool. How many people are in the office right now?
Alex 02:48
I think we've got four or five of the team in. We're about 18 people today, we'll probably be 20 in the coming months. So by the end of the year, we'll be about 25
Anthony 02:57
I have to ask, is the micro kitchen better or worse than the Google micro kitchen?
Alex 03:02
So Google micro kitchen is hard to beat. I think it still has the number one spot.
Anthony 03:06
Alright, fair enough. Fair enough.
Alex 03:08
It's not for startups.
Anthony 03:10
That's right. Know that, know that soft living, you have to, you know, buy your own fruit water. You know, I can't believe that you're the CEO of your company. What's it like to be a CEO?
Alex 03:25
It changes week to week. It's really whatever needs doing, I need to do. And I view my job first and foremost as making sure that we achieve our mission, that we're gonna give computers a sense of smell. And that is an incredibly tall order. It's a North Star that's far away. And we need to carefully choose our beachhead, and every step that we take on the way to the top of that mountain. So I view myself as a shepherd of that mission. Also, my job is to bring in the best people who are excited about that mission. And we need a really diverse set of talent, thought and background in order to achieve this. So I think about mission, I think about people all the time. That's almost 100% of my job.
Anthony 04:02
You know, I didn't hear the word deep once in your soliloquy. You’re not doing a good job of selling this man. You're the CEO, you're supposed to raise money. Nor did I hear the word disrupt anywhere.
Alex 04:13
So we're going to create a disruptive synergy using large language models in an AI first deep tech world. How's that is that where....?
Anthony 04:22
On the blockchain..
Alex 04:23
Yeah, I forgot - it's on the blockchain
Anthony 04:26
Re-enabled..
Alright, walking down the hall here
Alex 04:36
These are our new ingredients here. But also this is our ingredient fridge. This is kind of where we keep lots of stuff. But in here we've got our ingredients cabinet. So this is our vented hoods because this things can get kind of smelly. You put your head and give it a good whiff. It should be well ventilated, very few smells should be coming out of that.
Anthony 04:56
Yeah, it doesn't really smell smelly. It's kind of more or no kind of clean smelling soapy a little bit maybe?
Alex 05:05
How about that?
Anthony 05:07
Yeah, it has a kind of sweet, but also sort of alcoholic smell to it.
Alex 05:11
Yeah. So these are some of our reference compounds, which we use to train people to rate smells properly, because you in order to build a machine learning model that takes in the input of a molecule structure and predicts what it smells like, you have to have people that can tell you accurately what things smell like, so you have to train them.
Anthony 05:27
Right. So let me ask the big question to start us off here. How are you training computers to smell?
Alex 05:33
Very slowly and reliably and over a long period of time, but the essence of how machine learning has advanced into new modalities is, you have lots of inputs. So for images, that's actual pictures, and for audio, that's recordings of sound like the one that we're doing right now. And then you have human labels. So for images, it would be: “Is that a dog or a cat or strawberry in that image?”, and those are labels provided by people. So we need labels of what things smell like provided by people. So what that means is, you have to train people to learn how to associate words with odors, like cinnamon, or strawberry, or milk or grassy. And it turns out that with a couple hours of training, people can get really good at that. People can get as good at that as doctors are at reading medical images. And you have to use very specific protocols. And you have to watch out for people that have extra skill at associating words with odors, and then you know, gather more of those people onto your panels. But you can get high quality odor labels. And then you've got your x's and your y's, your inputs to the machine learning model and your outputs. And then we use a type of neural network called a graph neural network in order to learn how to associate the structure of a molecule to its smell. And that's the core engine inside of Osmo. And inside of that neural network is the thing that really is special about our technology, which is a map of odor, that's a really key part.
Anthony 06:51
Okay, so use a graph neural net. And just to make sure I'm understanding, the idea here is every compound you treat as a graph, and so the type of atom is a color on the nodes, and the type of bond is a color on the edge. And then you build a graph ML model, that then takes the labels and predicts human labels from the actual raw molecules themselves.
Alex 07:11
That's exactly it. That's the mechanistic part that's like the engine inside of the car, the thing that we had to build, and we chose to use graph neural networks to do this, but the thing that smell was missing was what the other senses already had, which is a map, right? So RGB is a map of color, red, green, and blue are three axes. And those three coordinates can describe any color and how to mix them. And low to high frequency. The Fourier basis is a map of sound, it's one dimension, that allows you to specify any sound or any song and share it or store it. But smell has not had a map. It's more complicated, though, so it's reasonable that we've had to wait for it. So while your eyes have three channels of color information, your nose has over 100 times more dimensions of odor information, 350 or 400, depending on how you count. So it was always going to be a complicated map, it was never going to be a map that fit on a flat piece of paper, or fit on a globe like sound or like vision. And we've had to wait for machine learning and artificial intelligence to build high dimensional maps that fit in software, as opposed to on a piece of paper. And that's exactly what we did while we were at Google Brain is we developed that map of odor technology. And that's the core engine that's allowed us to explore that map where every point on that map is actually a molecule, right, just like every point on a map of the globe is some piece of earth. Every point on our map of odor is a new ingredient. And some of them have never been smelled before. Some of them have never been synthesized before. So do you want to smell some of them?
Anthony 08:39
Yeah, let's smell.
Alex 08:41
So here is our set of best molecules. Alright, so this is one molecule that we placed on these beads so that it's easy for you to smell it. That's a molecule that we discovered using our map of odor.
Anthony 08:55
So what I see in the jar here is, like, almost looks like little Tic Tacs. Why is it putting on the bead make it easier to smell?
Alex 09:01
Those beads are really absorbent and so if you put a compound on those beads, it will stay on there. It'll be easy for it to transport and easy for you to evaluate it. But some of these molecules that we have are also in liquid form.
Anthony 09:14
This kind of smells a little bit like almost like chlorine at a pool. Kind of like a yes sort of medicinal smell to it.
Alex 09:24
Alright, this one is called 400, imaginatively named. What do you smell for that one?
Anthony 09:35
Little bit maybe kind of rusty maybe smell to it
Alex 09:39
You get like a leathery yeah leather.
Anthony 09:41
That's right. That leather is what it is. Yeah.
Alex 09:43
And you get any saffron.
Anthony 09:48
I do not get any saffron.
Alex 09:49
Interesting.
Anthony 09:48
I will confess I do not have a good sense of smell.
Alex 09:51
Okay, we hopefully we can have a machine for you just like you know, I needed glasses when I was younger in order to improve my vision. So Kim, our audio engineer, used to sell saffron. Does this smell like saffron?
Kim 10:05
Oh my gosh, that's exactly saffron.
Alex 10:08
Which part of the saffron flower is it?
Kim 10:12
It's the stigmas, but more towards the white part.
Anthony 10:15
Wow. Color me impressed. Okay!
Alex 10:18
So that's one of our molecules here.
Anthony 10:20
Okay, so if I recall from my medical school neuroscience class, you have things called odorant receptors, right? And these are molecules that sit on the surface of neurons. And each of them is able to bind to a molecule. Is that right?
Alex 10:35
Yeah, those neurons are actually one of the only two parts of your brain that leaves the skull. One’s the hypothalamus that dips into your bloodstream, and the olfactory sensory neurons start in your brain in your skull, they poke out through little holes that lead into the inside of your nose. And that's your brain that's actually directly touching the world when you're smelling things. So these neurons express one type of receptor. So the surfaces of these neurons have little tiny fingers that reach out of the brain, into the mucous layer inside of your nose. And those are called cilia. And those silly are covered with receptors and just one type per neuron, that was actually a huge discovery that in part led to a Nobel Prize by Linda Buck and Richard Axel, the one receptor one neuron rule. And those receptors are in the mucous of your olfactory epithelium, which is like a postage stamp sized piece of flesh that is really sensitive to chemicals, that's the part of your brain that's outside of your skull that can actually smell - it’s like the retina for smell. And this epithelium is covered with different kinds of olfactory sensory neurons, each of which are expressing one receptor, and there's about 400 different receptors that we have. So this is in pretty big contrast to how our eyes work, where there's just three kinds of color receptors that roughly correspond to red, green, and blue, we have 100 times more dimensionality in the olfactory system. And we don't actually know what a lot of those olfactory receptors look like. Although one has recently been crystallized bound to a ligand, which was a huge breakthrough. And I think we can expect more. But we don't really know what their tuning curves are. So for any given receptor, we don't know what it binds to, for the most part.
Anthony 12:15
And so does each olfactory receptor bind one molecule, or can it bind many molecules?
Alex 12:23
So these are interesting receptors. So normally, we think of molecules and receptors as a key and a lock. And that receptor is designed for just one type of molecule. Olfactory receptors, we think by design are really sloppy, they can bind a lot of different molecules, they can detect a lot of different things. And it's very rare that we see olfactory receptors that bind just one type of molecule. So they bind a lot of different stuff.
Anthony 12:49
And so let's say the two different molecules bind to the same olfactory receptor. Can we distinguish those molecules by scent? Or do they smell identical? The
Alex 12:59
The olfactory system doesn't work one receptor at a time, it's a portfolio sensing effect. So when you think about what Something smells like, it's never just one receptor at a time where you have to think of this. And that's what makes it so hard to study. Sometimes you have to look at all 400 of them in order to understand how we're going to perceive it.
Anthony 13:16
Okay, so let me make sure I got this right, there's actually a mini to mini map between molecules and odorant receptors, one molecule can bind many odorant receptors, one odorant receptor can bind many molecules.
Alex 13:28
Absolutely. And that's the magic. And I think the power of the olfactory system is it's designed to detect such a diversity of molecules. And it's come up with this very strange, scattershot strategy for doing it. And that seems to be a strategy copied across evolutionary time. Every animal that has a great sense of smell has this pattern of a whole bunch of diverse receptors that can detect a whole wide variety of molecules.
Let me pull out another one. This is interesting. So in the world of pharma, you'd call this a hit expansion. Do you know what a hit expansion is Anthony?
Anthony 14:00
No.
Alex 14:01
So when you find a good molecule, and you're developing a drug, it doesn't always do everything that you want it to do. So you need to modify the molecule a little bit to make it more potent, or to make it more safe. And so what we did is we found a molecule that had a mild red berry smell. I'll give you a chance to smell this first.
Anthony 14:21
Yeah, smells like red berry.
Alex 14:24
It's kind of faint, though, right? Yeah. So what we did is we said, Alright, this is a good start. But let's see if we can improve the potency of this molecule. So here is our hit expanded version. And we did this just a few weeks after finding the original one.
Anthony 14:41
Well, that's way more pungent. For sure.
Alex 14:44
Yeah. So to me, it's a much bigger, rounder, red berry, it's got like a clear character like you can almost it's you can almost name the fruit - kind of like a grape or maybe a little bit of a blueberry. So this is the AI platform that we use in the map of odor that we use… at work, right. So we start with a good molecule. And then we search around in our map of odor in order to find something that's even more potent, that's even clearer from an olfactory perspective. And of course, we put all of these molecules through really stringent safety testing. So they're all at different stages of that process. And of course, we'd never release a molecule that wasn't perfectly safe. And that also wasn't good for the environment, which is a problem with a lot of fragrance ingredients. These ingredients, when they become popular are made by the tonne, right, there's a lot of it, it gets into a lot of shampoo, like a hand soap, a lot of fine fragrance. So the real thing to worry about from a safety perspective is not necessarily what it does to your skin, which is important. But it's more important to think of what it does to the environment, because this is all going to get flushed down the drain, it's going to make it into our waterways and into our soil. And a really good molecule, a biodegradable molecule will break into pieces that can be recycled into nature, no problem. A problematic molecule will stay in its current form or won't break down, or it will break down into something harmful. So we want to avoid that. So we're very, very careful about that when we design these molecules.
Anthony 16:02
Is that totally a chemistry process? Or is there also machine learning to predict toxicity as well.
Alex 16:09
We use machine learning for all predictive aspects of the chemical development process. So we try to get the molecule as right as possible in the computer before we synthesize it. And then of course, we have to test it to make sure that we were right. Hey, Mike what’s up?
Mike 16:21
Just to barge in - in about 45 seconds, there's going to be something kind of cool on the one at the end.
Alex 16:28
Let's go. Yeah.
Anthony 16:29
All right, let's check it out.
Alex 16:32
All right, so we've got a Gas Chromatograph Olfactometer, which is a blend of an oven, which has got a very, very long racetrack, a very thin, long racetrack where molecules are zooming from the sample where they're all jumbled together. And they're passing out of this oven one by one. So you can think of it like, like a marathon race where all the runners fast and slow, fat and skinny, are together at the starting line. And then as the race goes on, they actually separate and they cross the finish line one at a time. So what we're looking at on the screen is basically the amount of molecules that are coming out of the end, that are all running that race at the same time. And so the faster ones have already come off, and the slightly slower ones are coming off live. And when they come off of this GC oven, Anthony's smelling them. So he's got his nose at the end of a Gerstel GCO port. And he's sniffing actively, there's new molecules that are coming off. So far, he's not smelling anything. I feel like I'm announcing, like a horse race or something like that.
Anthony 17:28
Yeah, so far, I haven't smelled anything, like maybe hints of something like every once in a while, I'll get like maybe a little bit of floral scent, or maybe a little bit more of like an alcohol scent. But it's pretty faint.
Alex 17:39
Yeah, so this is a sensitive instrument, and what will come off will be faint. Right? So those little impressions that you get, are you smelling the individual components of a complicated mixture of the fragrance oil, so you're actually smelling the kind of skeletal pieces of a fragrance oil that have been exploded apart, as opposed to them all mixed together again.
Anthony 17:57
And t's just basically based on their, their boiling point, if you will, in terms of the point at which they evaporate and go free, right?
Alex 18:04
Yeah, so the molecules that are lighter and have a boiling point, which would cause them to volatilize, or come out of sample faster, those are coming out of the oven faster. And the ones that like to stick into the oven, or the long capillary tube, you know this 20 or 30 meter long tube, those come out slower. And there's lots of different kinds of columns. But in general, you can think of molecules coming out first are smaller or lighter in some way, and molecules coming out last as being larger or stickier in some way. But you know, there's, there's different details and different, different ways that you can set up the system to work.
Anthony 18:35
So is there like, I guess I want to say the equivalent of bandwidth, where there's a heaviest molecule that you can smell and a lightest molecule that you can smell, or does it not work that way?
Alex 18:46
No, that's totally correct. So the largest molecules that you can smell are the ones that can just barely lift themselves off the ground, actually, to get into the air. So it's like, imagine an airplane that's just too big to take off. And it's basically a really big car at that point. Molecules that can't make it out of solution into the air don't have a smell. And there's a limit to the size of a molecule that can do that. And then of course, there's molecules that are too light that actually can't get back into solution inside of your nose. So like, you'll never smell, CO2, you will never smell hydrogen, you will never smell oxygen.
But you can smell molecules that are just a little bit heavier than that. So there's a, there's a left and a right limit to what you can smell. There's molecules that can be roughly the same weight that can have dramatically different odor potencies. So if you add a nitrogen or a sulfur to a molecule and take away a carbon, you'll get something that smells like fish or like garlic, and it will be incredibly potent, whereas a molecule without nitrogen or sulfur will often be less potent. But it's difficult to predict. That's essentially why we need machine learning. It’s because the relationship between the structure of a molecule and what it smells like is really complicated. But we can learn it from data.
Anthony 19:48
The sense I'm getting for you is that this is a very nonlinear problem. Like small changes can have big effects on the smell and moreover, smells interact with each other. Is that fair?
Alex 19:58
Absolutely. You can go from roses to rotten eggs by moving around a couple of electrons instead of a molecule, I mean, there can be really, really, really big changes to how we perceive a molecule with tiny changes to the structure of the molecule. In medicinal chemistry, you'd call that an activity cliff. And that's kind of the rule as opposed to the exception in odor there's really large changes that can occur in how we perceive molecules with small structural changes.
Anthony 20:22
So what is the point of this in terms of .. I have a complex mixture and then I break it apart into its component smells? Like, why do you have this machine? What's the experiment you're running here?
Alex 20:33
So when we make new molecules, we want to make sure that what we've made is actually what has a smell. So what we need to make sure is if there's multiple components inside of a given oil or inside of a given ingredient that we're making, we need to make sure that the main ingredient is the one that smells the way that we think it does the way the whole mixture smells. So we use this to pull apart the entire mixture to the extent that there are other ingredients and verify with our noses, which are still the most sensitive chemical detector instrument in the world. We need to use our own noses in order to verify that we've made what we thought we've made. So this is a critical quality control instrument for us.
Anthony 21:09
And I assume that the reason why this machine exists is that basically every company that's doing flavors or fragrances needs to run this experiment to disentangle their molecules. Is that fair?
Alex 21:20
Absolutely. This is a non-negotiable part of a quality control pipeline in a fragrance company.
Anthony 21:26
Okay, so then let's go back to how you trained your models. You said that what you need to do is take an individual molecule, and then have humans label it according to what it smells like. So talk me through a little bit more of this process. What's your human vocabulary to describe the scents, how many humans were actually labeling samples? How many samples have you gone through?
Alex 21:49
So we've had multiple phases of building this training dataset. But I think the way to think about how we normally do it is to imagine a Crayola crayon box with 64 different colors in it. Except we don't have 64 colors, we have 55 odors, and each of those odors, instead of being a colored crayon, is a little vial with a reference compound in it. So when we say fresh cut grass, we have a vial that's filled with a molecule called six three hexanol. And that's the canonical reference. And that's what allows people to learn the association between an odor and a word. And it turns out that you and me, as opposed to, you know, very highly trained master perfumers. You and me as non experts can learn this lexicon, this Crayola box of odors in a couple hours. And once you've learned that association between words and odors, you can go on and label new things. And it turns out to get statistically reliable data, you need a panel of 10 to 20 people. Once you've got 10 to 20 people labeling the odors, you can really count on those labels as being able to be used later on in the context of machine learning.
Mike 22:56
There’s something coming out - did you get anything?
Anthony 22:57
Yep, it's still kind of a cinnamony kind of smell to it. Yeah, it's kind of over now.
Alex 23:05
And on the screen, the traces declined down towards zero. So that makes sense.
Why don't you smell the actual mixture that you smell the pieces of?
Anthony 23:15
Yeah, so this has like, this smells like a very, it reminds me of something but I can't place it. Like there's a mixture of kind of a bit sweetness. But also a bit sort of astringenty. Yeah, what do you smell?
Alex 23:40
It's a blend of a lot of stuff. So I've got something kind of musky, something soapy, aldehydic. I think from a distance there's also like a jasmine like a Narcissus Funk, which is like some flowers smell a little bit and animalicky.
Anthony 23:52
When you say animalicky, what does that mean?
Alex 23:54
I mean, like a kind of a stinky part of an animal.
Anthony 23:58
Very technical!
Alex 23:58
Very literal about that. Yeah. So is there anything in this that you, any ingredient that you smelled on the GCO that you can detect in the whole mixture?
Anthony 24:08
A little bit, the soapiness, that was the one that came through on the GCO.
Alex 24:12
Got it. That's awesome. So you were able to pick out a single ingredient in a mixture of many, many ingredients, because we split them apart on the GCO. That's pretty awesome. Some of those ingredients are going to disappear in the mixture and have really strange nonlinear effects. Because when you start to mix multiple molecules together, they can create surprising smells that don't really smell like the sum of their parts. So you know, you might smell something very distinct on the GCO, but it might be invisible in the mixture, which is exactly why you need this instrument in order to be able to pull these things apart, identify the constituents. If your goal is to remake that mixture or to identify contaminants, you have to have an instrument like this.
Anthony 24:45
Actually, you know, a question for you is in the world of metabolomics on let's say human blood. A very classic problem is that you can get peaks on the mass spec machine and have no idea what the underlying metabolite is. Is it the same problem here where you're seeing peeks on the machine and you don't understand it, you don't know what the molecule is underlying it?
Alex 25:05
So I've heard different numbers that you know, up to 80% of the molecules that you see circulating in your blood when you analyze it with these machines are unknown. It's kind of like a dark metabolome. Right? And it drives me crazy, like, why don't we know what those things are? Right? So it is the case when you look at the volatile organic compounds in plants. So when you go out into nature, and you pull out some essential oil, and you analyze it, you're gonna see a lot of things that you don't know, when you analyze modern perfumes, most of the ingredients are synthetic, and most of the ingredients are known and they're regulated. And so it's a different story when you're understanding how to reverse engineer a fragrance, because it's made by a person as opposed to made by a genome. But sometimes people use essential oils and these fragrances. Fortunately for us, a lot of these essential oils have been analyzed very, very closely in the past. And so we'll know what the major constituents are, there's still going to be a tonne of stuff that we don't know. But we'll know what the, I guess the key chords are. So there might be 100 molecules in lemongrass oil, but we'll know that there's 10 that are important for its olfactory profile, and could be less could be more depending on the essential oil. But there's still a lot that's unknown out there.
Anthony 26:16
Alright, so let me go back to the non linearity. So one of the things you said earlier that I found fascinating, is really small changes in a molecule can have big changes in its scent. structure doesn't necessarily correlate to function. So if molecules don't cluster, by their shape, are there ways that they do seem to cluster together with respect to their scent?
Alex 26:40
Let me pop up a level. And let's just think about what scent actually is. But first, let's talk about a system that we understand vision. So every animal that sees in color has receptors that roughly correspond to red, green, and blue, some have an extra in the ultraviolet, some have an extra in the infrared. That's not surprising, if you think about where light comes from, which is the sun. And the majority of the energy that the sun outputs is actually in that range that's covered by red, green, and blue. And so the sun is forcing evolution to adapt eyes to cover that range of the light that's on earth. Now, what about the sense of smell? What's the equivalent of that prior for smell? And the answer is, we've been all smelling the same air for the last 500 million years. And that air has been filled with molecules produced by plants and by insects and by animals. And those plants, insects and animals produce smells from internal metabolic processes, where their insides get to their outsides, right, either they shed skin or a little bacteria in the soil produces off gassing, or something dies and it rots. The inside is always getting to the outside. And so the prior over smell isn't like light from the sun, it's the metabolic processes inside of living things that get to the outside, that's what the air is filled with. The air is filled with life. So the prior over what things smell like should be the processes of life. And the processes of life are enzymes that take starting material starting molecules and turn them into some other molecule. And in all of our cells, there's this web of metabolic activity of molecules being transformed by an enzyme into another molecule that's fed to another enzyme transformed to another molecule. We call this web a metabolome, which is the set of all transformations that can occur inside of a living thing. So we thought maybe the metabolome, the relationship between all molecules produced by life, maybe that can inform what things smell like. So we did this experiment, where we downloaded all of the metabolomes that have been curated about all the organisms that have been studied - all of them. And we looked at the relationship between molecules on this metabolome. So let's say take molecule A and molecule B, that's found inside of the same living thing, how many enzymatic transformation steps does it take to go from A to B, it might be one, it might be five, it might be 10. That's a metric that indicates how metabolically close two molecules are. Now what we can do is use our very accurate model of scents to predict how similar those two molecules smell. So now we have two numbers. We've got metabolic similarity derived from real data, and the perceptual similarity derived from the first accurate model of odor. And then you can plot them against each other. And what you get is a nearly perfectly straight line.
Anthony 29:35
Wow, this is an amazing result. Let me make sure I'm getting this right. You're saying that if one metabolite gets broken down into two metabolites, then they're more likely to smell similar to one another. And so what our nose is really picking up on is the metabolic processes of life.
Alex 29:54
Absolutely. And there's lots of examples where you can just point to it. So the things that makes trees smell woody, are breakdown products of their bark. The thing that makes saffron smell the way that it does are breakdown products from the dye that makes saffron red. There's all kinds of relationships between what makes a living thing inherently itself structurally or colorful, that relates it directly to its smell. So things that are breakdown products inside of a living thing that are nearby metabolically, of course, they should smell similar. It's what makes that living thing itself. It's what makes it its own characteristic self. And that shows up in scent as well.
Anthony 30:33
Okay, so then there's another non-linearity dimension that you mentioned that I want to understand, which is that a mixture of N molecules isn't a linear superposition of each of their individual scents. So I'm trying to understand why that's the case. Because if I have one odorant receptor per neuron, I would have thought that, it would just end up being kind of a linear summation, what's going on?
Alex 30:57
So imagine all of the 400 olfactory receptors as keys on a piano. So you have a 400 key piano. And a molecule is somebody playing some number of those keys. And it could be one key, it could be 50, it could be all of them, right? to different levels of intensity. So you know, you're banging the keyboard, a molecule bangs, the keyboard of our olfactory system. Now, what happens when you add a second molecule that wants to play some of the same keys? The keys already pressed?
Anthony 31:26
Oh, I see. So the molecules are competing with each other in activating the olfactory system.
Alex 31:32
Exactly. So if you're trying to put a key in a lock, and there's already one in there, it's not going to work in the olfactory system. It is the only perceptual system in our body where this kind of competition happens at the periphery, in the sensory organ. There's all kinds of optical illusions that have a kind of a competitive nature, like you see one thing as opposed to the other, but that happens computationally in the brain, it doesn't happen physically in the eye.
Anthony 31:59
All right, Alex, that tour of the lab was absolutely fascinating.
Thank you so much for that.
<<Music>>
So now we're getting to one of my favorite parts of each of these episodes, which is the Hammer and Nail section. So you know, as a reminder to our audience, we usually take time at the end of each episode, going back to our roots in a series of meetups that you and I ran in Boston many years ago, to discuss a big problem, the nail and possible solutions to it, which are the hammers. And this is always inspired by what we just heard from our guests. So you know, Alex, you just talked us through the journey of being a researcher, to being a manager of people to now being a CEO trying to build a business. You know, where does this all go? What's your, your ultimate vision and Northstar? And how does that relate to kind of hammers and nails?
Alex 32:48
It's a personal one. I've been obsessed with digital olfaction and machine olfaction and olfaction, in general for a long time. And I've been learning as much as I could about how smell works, and how smell can affect our lives. And one area that I found to be really fascinating over time, is the area of scent for diagnosing diseases or telling how well our bodies are functioning. So smell is a diagnostic.
And we've known for a long time that dogs can smell cancer, dogs can smell COVID-19, bees can smell COVID-19, rats can smell malaria or landmines. I mean, the list of detection tasks that animals can do, that are out of range of what our mobile computers can do is just astounding. And if computers could do these kinds of things, people would live longer lives full stop. And it became personal for me when I was in the middle of graduate school, and my dad got very sick. And he ended up after a biopsy, we found that he had brain cancer, and he had multiple tumors in his brain that might have been growing for a month or might have been growing for years, we will never know. And I took off time from school to care for him and he passed away six months later. And there were two parts of my brain or personality. There was the part of me that was a son that took care of them. And then there was the part of me that was a scientist that had put that part of my life on pause. And it took me a while to recover from that and for those two parts of my life to come back together again.
And when they did come back together again, a couple things happened. One is I felt a bit ashamed that as a neuroscientist being trained at one of the greatest programmes in the world, I hadn't reached out for help. I hadn't asked any of my neuroscientist friends or professors or medical researchers to help me figure out if there's any frontline treatments that could have helped him live longer. I didn't do that. And that's something I'll always carry with me.
The other thing that I started to do was I realized that this world of olfaction, and his illness actually had an intersection, which is that animals can smell cancer. Animals can smell specifically brain cancer, although the evidence is scattered. It's an indication that when something is happening on the inside of our body, it's killing us, the inside gets to the outside somehow. And what gets to the outside can be detected by animals, or hopefully, by machines with a very sensitive sense of smell.
If we had caught his disease earlier, it's possible that maybe we could have done something about it. And if not, for him, having that diagnostic tool might be useful for other people who get sick in the future. Because if we can detect a disease early, we can start to build treatments against it. So it's, it's a very personal thing for me that we haven't yet really embarked as a society on figuring this problem out, we don't really know the molecular content of our breath, we don't really even know the molecular content of our blood…over half, sometimes up to 80% of the molecules that you see circulating in our own bloodstream are unknown, at least one of those is going to be a signal that tells us whether or not we have some disease, or whether or not we should make a diet change or whether or not we should get more or less of a certain type of exercise, that signal that's completely untapped. And we've started doing this for blood with things like the Framingham study, where we've studied the contents of the circulating factors in people's blood and related it to cardiovascular disease, that gave us incredible insights. I mean, you as a cardiologist know better than anybody how important that study was.
Anthony 36:56
You know, this is incredibly moving, and incredibly powerful at the same time. Let's riff for a second on what a Framingham for breath would look like. How would you go about collecting breath samples? I mean, how do you store them? What do you do to actually get all the metabolites out, like, walk me through the anatomy of a breath of air?
Alex 37:20
Yeah, it's, it's unfortunately, not as well studied as we want it to be, there is a field of researchers that study breath in, they're incredibly motivated and incredibly intelligent. But the field is so much smaller than other larger fields of biology, like genetics. So here's how you look at breath. And here's how you store it. So there's three pieces to an exhalation.
The first is the light gas like carbon dioxide, nitrogen, oxygen, you can just measure the amounts of those and then throw the information away. Then there's the heavier gas, these are the things that actually have a smell. So like when your breath smells fruity or bad, this phase of breath is where those molecules live. That's where the scent of breath is. And then there's the aerosol phase, there's little droplets that leave your mouth that actually can contain things like viruses. That's how COVID-19 spreads, it hitches a ride on the aerosol phase of breath. And that's where bacteria live, DNA, RNA proteins, I mean, these aerosol droplets are just full of signal.
And every breath that you exhale, has over 1000 unique kinds of molecules in it. And what you exhale is what was previously in your blood, your blood is in constant contact with your lungs, and exchanging information. So here's how you store it. For the aerosol phase 10 minutes of breathing produce about an Eppendorf tube, like a milliliter or so of liquid, you can store that on the cold chain, just like you would blood. So just store liquid breath. And then for the light gasses, just measure them, there's simple tools for doing that. And then for the heavier gasses, there's a couple strategies, but that's where the most technical developments required, you can use little fibers called beamy fibers or twisters that can absorb the heavy gasses, you can then store them and then you can kind of burn them off and analyze them in the same instruments that you saw in the laboratory earlier. So it's still being developed, but at least we know how to analyze the earliest kind of forms of breath, and we know how to store the liquid phase.
Anthony 39:18
Okay, so then once you actually can store the breath, is the next step to do mass spec on it, or are there other assays you'd want to do as well?
Alex 39:27
At the very least you want to do mass spec. And you could maybe even do that at the moment of collection. Although the unit economics of how you'd run the study, you're gonna determine what the best way to do that is. But once you've got the metabolomics down, which is going to be no small feat, that's going to be hard because some of the molecules in your breath occur at vanishingly small amounts, and you need very sensitive instruments to pick them up. But then you should start looking for DNA and RNA and proteins because there's life that you're breathing out in the droplets that you're exhaling, and we should know what's in them.
Anthony 40:00
And so then a question for you too when you think about let's say diagnosing Parkinson's or cancer, is it actually your breath that you want? Or what about metabolites that are coming off of your skin? And could you even imagine, like, doing things like skin biopsy to try and get that information?
Alex 40:16
You're completely right. When we say breath, what we should really say is exhalations of the body. Because your skin is emanating smells, your skin is also producing waxy substances or oils. And actually, that's where Parkinson's disease is diagnosed from by smell. So that's one example that's been run all the way to ground where it turned out that there was a nurse in the UK, who discovered that she could smell Parkinson's disease. And the way that she did it is she worked on a ward where she was smelling people with the disease, and she noticed that her husband started to take on this musty smell. Her husband was later diagnosed with Parkinson's disease. And a medical group put it to the test. They said, Alright, here's 10 people with Parkinson's disease. 10 People without, we had them wear a t shirt for a couple of days. Can you smell Parkinson's disease? And she got 19 out of 20, right? And she actually said, “No, I'm pretty sure I got 20 out of 20 right?” They said, “Nope, this person doesn't have Parkinson's disease.” A year later, that person comes in diagnosed with Parkinson's disease. Right? They tracked the signal she was queuing in on to the middle of the back with it admits a substance called sebum, which is like a waxy excretion we all have, it sounds gross, but we all we all have it. In that substance, there was a re-regulation of molecules that was only happening and people with early Parkinson's and advanced Parkinson's disease, and those molecules have a smell. That's how she was able to diagnose it.
Anthony 41:50
So why don't we use it clinically?
Alex 41:53
Anthony, I don't know how to answer your question. You better than most, and your partners at GV understand just how complicated it is to make sure that a diagnostic is useful and safe and also good business, it's reimbursable. We're still in the earliest days of the science. So let's be honest with ourselves, this isn't something that we can, you know, turn a switch on right now and save lives. There's a lot of basic fundamental work that needs to be done. We just need to start doing that work. And we need to understand the smell of disease with the same rigor that we've done for liquid biopsy or for other aspects of metabolomics. The science is early, but we've got to get started.
Anthony 42:31
And so you know, walk me through your vision for the Human Breath Atlas, how many people? What kind of people would you want? Would you want to enrich for disease? Would you just want to take all comers? Like, what would be the design of the study?
Alex 42:44
Well, there's already a group of folks that are working on this under the name of the Human Breath Atlas, they're very talented breath researchers. I think we need to think about a biobank of breath at the same or greater scale as the Framingham study or the UK Biobank. I think we don't know what we're looking for. And when we first started to sequence the human genome and try to associate single nucleotide polymorphisms, or mutations in genes with disease, we get overwhelmed with a diversity of genomes, right and overwhelmed with a statistical problem of so many inputs, so many points in the genome that could change and so many phenotypes that could be changed by them. And we invented new statistics in order to make reasonable sense out of that, but we needed large numbers in order to do that. There are so many different molecules that the body can make. You can look at the human metabolite database, you can look at metabolomic studies. 10s, or hundreds of 1000s of unique molecules, right? We're in the regime of complexity of the genome, probably not reaching 3 billion base pairs like the genome has, but you know, same order of magnitude where we need to think in terms of very large biobanks. So we're going to need 1000s or 10s of 1000s of subjects tracked longitudinally over long periods of time, because we're looking prospectively, we don't know what we're going to find, just like the Framingham study didn't know it was going to discover statins. We don't know what we're going to find. But the point is, we need to start looking.
Anthony 44:11
Excellent. That seems like an incredible intellectual journey and one that I'm really excited to see you take over the coming years.
Alex 44:17
Thanks, Anthony. I know that we're going to do it together. You've been an incredible help and supporter on this journey. So far.
Anthony 44:23
The feeling's mutual, my friend.
Alex 44:25
I hope that we can do this shoulder to shoulder
Anthony 44:26
You too.
Alex, thank you for joining me as our guest this week.
Alex 44:29
Thanks, Anthony.
Anthony 44:31
Many thanks also to Kimberly Jung, CEO of Blanchard House, who recorded this episode at Osmo.
We'll be back next week to ask Professor Jim DiCarlo - what happens when you use artificial intelligence solely for the purpose of understanding human vision? We'll get to directly explore what we can learn about ourselves as humans from AI.
We'd love to know what you think. So please write to us at theoryandpractice@gvl.com or tweet @GVteam.
This is a GV podcast and Blanchard House production. Our science producer was Hilary Guite, executive producer Duncan Barber with music by DALO.
I'm Anthony Philippakis
Alex 45:13
I'm Alex Wiltschko
Anthony 45:15
And this is Theory and Practice