Computational Neuroscientist Dr. Thomas Reardon on Communication between Minds and Machines
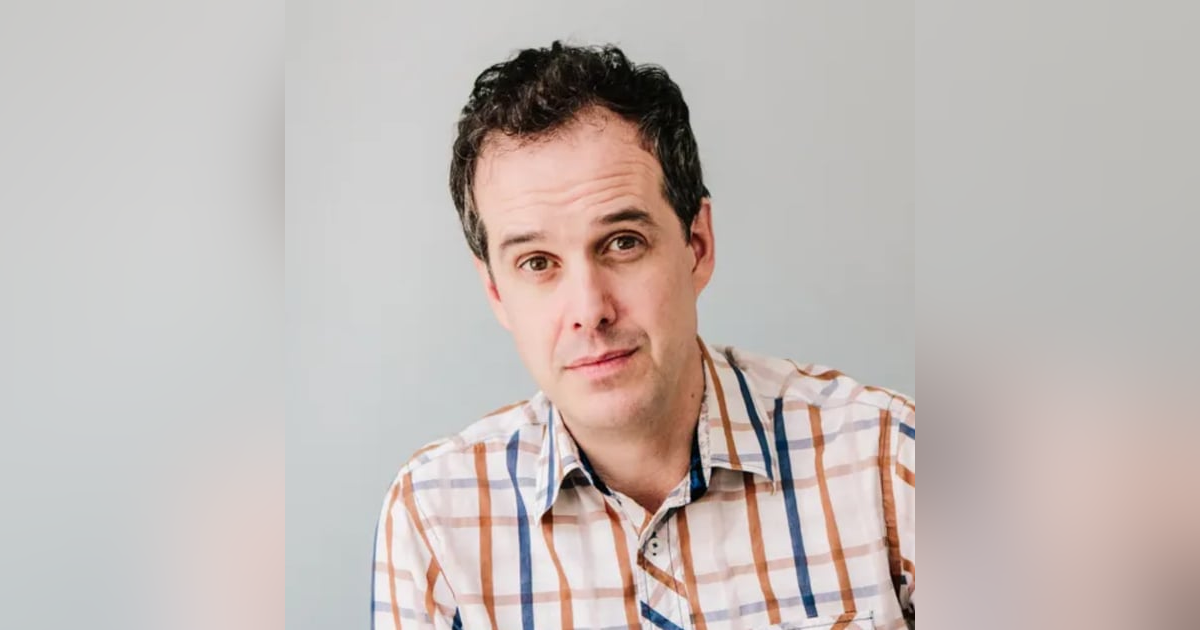
In episode 3, we explore how humans will control computers in the future with Dr. Thomas Reardon. Dr. Reardon founded CTRL-Labs and is an early pioneer in exploring the relationship between humans and machines. Now at Meta’s Reality Labs, Dr. Reardon continues his work on non-invasive neural interfaces that detect activity in the human nervous system.
On Season 4 of Theory and Practice, Anthony Philippakis and Alex Wiltschko explore newly emerging human-like artificial intelligence and robots — and how we can learn as much about ourselves, as humans, as we do about the machines we use. The series will delve into many aspects of AI: from communication to robotic surgery and decision-making.
In episode 3, we explore how humans will control computers in the future with Dr. Thomas Reardon. Dr. Reardon founded CTRL-Labs and is an early pioneer in exploring the relationship between humans and machines. Now at Meta’s Reality Labs, Dr. Reardon continues his work on non-invasive neural interfaces that detect activity in the human nervous system.
Dr. Reardon explains how these systems encourage co-adaptation between man and machine. This human-computer interaction is core to understanding how human-like AI is changing humanity.
Alex also describes the complexity of haptics — how computers try to relay the depth of human senses. He explains why understanding touch is essential for the future of human-like robotics.
Anthony 00:04
Welcome to GV Theory and Practice. This series is exploring what it means to be human in the age of human-like AI. I'm Anthony Philippakis.
Alex 00:12
And I'm Alex Wiltschko.
Anthony 00:15
In the first episode in this series, we've had a fascinating insight into the safety guardrails for implementing AI in healthcare. Personally, I took away from it just how essential human individual values are for building trust with AI machines. And in our last episode, we learned how important empathy is in all communication and that computers can sometimes do this much better than us. But today, we are flipping the focus of our discussion, where instead of talking about how computers communicate with us, we're going to talk about how we communicate with computers.
Alex 00:49
Yes, at its most basic, we type commands into a keyboard, the computer listens. And more recently, we can speak to our gadgets and tell them what to do. But they don't always take note, Siri does not always understand what it is that I'd like her to do. So what if you could make things easier for everyone, by allowing your whole body, your muscles to talk to computers, for instance, a wave of your hand or twitch of your little finger translates into your lights turning on in your house or kitchen turning on and starting to cook your dinner, doors would open and your favorite music would come on, move your thumb and your new messages would be read out loud to you.
Anthony 01:27
It sounds amazing, I must say my friend. Let's go on and meet our guest. He was the first person to start building Microsoft's web browser, the Internet Explorer, which was the world's most used browser in the early 2000s.
Alex 01:39
And I have fond memories of Internet Explorer, that was the beginning of the internet to me. I have very clear memories sitting at a CRT monitor with Windows NT 4.0 installed and using Internet Explorer to basically start to access the world's information. And our next guest is responsible for a lot of that experience.
Anthony 01:58
You know, he's actually also had a fascinating career since then, when he made IE he hadn't yet graduated from college, went back to Columbia, majored in classics, but then caught the neuroscience bug and did a PhD at Columbia, working on peripheral motor neurons. And I think it's really fascinating to think about how this combination of neuroscience and software engineering has really traced through his whole career. He'll guide us through understanding how we will be communicating with AI and robots in the future through our muscles in our forearms.
Reardon 02:28
Hey, my name is Thomas Reardon, and friends and colleagues call me Reardon. I'm a computational neuroscientist, and I lead the neural interface work at Meta Reality labs.
Anthony 02:44
Thank you so much for joining our show today.
Reardon 02:45
Thanks, Anthony.
Anthony 02:46
You know, in the first two episodes of this season, we've been talking a lot about the input that the computers can give to humans. But one thing that I find amazing about your career is that you've actually flipped this paradigm on its head. And for the last decade or so, you've been studying the ways that the output from our nervous system can be better used to control computers and machines. So how did you start going down this road and what got you excited about it?
Reardon 03:12
You know, we started CTRL-labs back at the end of 2015. And I wouldn't say that it was started with such a clear mission statement of working on human output. Rather, it was a set of neuroscientists who are really obsessed with trying to do something that wasn't based on wherein where most academic neuroscience is based today, which is neuro pathologies. We wanted to do things that were kind of in the realm of neuro augmentation, and maximizing what neural gifts most people already have.
So having started on that path, then we started to think about, you know, where is it that people are not able to leverage their, their inbuilt magical nervous system as much as they ought to be able to, because of this imbalance between humans and machines, namely, that machines sort of shout at us all day long, in a very high bandwidth fashion. But we aren't able to shout back at them in a high bandwidth fashion, we receive all of that into our nervous system, but we're actually not able to generate much back out of our nervous system, because we're slowed down by movement, just actual movement, moving my lips, moving, you know, my lungs, and my pharynx, etc, to communicate verbally to use my hands to communicate via a keyboard or a mouse. All of this is a very, very low bit rate, compared to what a machine can actually get back to us. So we just kind of set ourselves up kind of in an abstract way to work on this problem of the imbalance between humans and machines. And in this input output imbalance,
Anthony 04:50
Actually, I'm kind of curious, is there actually a quantification of the bandwidth into our brain from all of our senses, and then the bandwidth of our output? Like do we actually have numbers in terms of information theory.
Reardon 05:02
I mean, people have tried to write down a number for this, I always think these things are a bit, you know, hard to verify. But there are estimates of between six and seven orders of magnitude difference in terms of, that's why that's, you know, a million to 10 million times difference. And that's because really, your input system is massively parallel. And while your output system is somewhat parallel, it's incredibly slow, because in the end, all you're doing is turning muscles on and off. And that process is necessarily slow. You have millions of neurons that are directly taking input from the world, and particularly the ones like proprioceptive neurons that we don't think much about, in which your brain is sort of saturated by these at the first order, meaning your brain extends sensory neurons out throughout your entire body, and you're taking in information at a unbelievably parallel and fast rate. But all of that output, again, is just going through, you know, 200 muscles in your body very, very slow compared to the number of neurons that are taking in information.
Alex 06:03
One thing I wanted to pick up on Reardon was what you mentioned before, which is augmenting the magic of the nervous system, this incredible swiss army knife that we have, which is our bodies, our nervous system that's given to you know, billions of people, we focused on this podcast on the value that AI can bring to improving human health and disease, but we haven't focused as much on augmentation. Right. So your focus has been on virtually the whole population, right? So for example, Internet Explorer reached around, you know, billions of people. So why do you think targeting whole populations first, rather than diseased populations first is the right approach?
Reardon 06:40
You know, this is just, I guess, something of a philosophy that I shared with my co-founders that we will actually be able to move the science forward faster. And I suspect Alex, you'll actually share some of this with me. We'll move the science forward faster, by trying to work on scale problems first, and then partnering with clinical practitioners who can then adapt the scale discoveries, to clinical populations, to people with different kinds of neuropathy, etc. I really feel like a lot of neuroscience does get slowed down by this kind of clinical first pathology, first approach to understanding decoding the brain. We thought by using effectively at heart machine learning at very, very high scale, we would learn things about the brain that are hard to learn in this kind of bespoke single experiment at a time approach that academic neuroscience is mostly centered around.
Anthony 07:42
When you think about trying to navigate the output of the human brain. What are the tools that you're using? I mean, is it physically controlling devices? Is it EMGs and ECGS? What are the main tools you use?
Reardon 07:55
Yeah, so our main approach is invested in what we call non invasive neural interfaces. So this is being able to detect activity of the nervous system without effectively perforating the human body. So we're not drilling into the skull, we're not trying to insert anything inside of you. That leaves us with a few different techniques, you named a few of them. EMG is one electromyography, which is listening to the activity of the neural response, the electrical response of muscles to neural inputs. Another one would be EEG, which is kind of at a distance trying to listen to the large electrical waves that hundreds of 1000s of neurons in, say, cortex generate. Another one might be MRI, or we call functional MRI, which is even bigger, maybe looking at the activity of millions of neurons, and looking for metabolic signatures of activity. We are focused on EMG, which allows us to actually listen to individual motor neurons at the currency of the nervous system, which is called an action potential or spike. And that's one of the things that we're kind of the most invested in is this idea that you don't actually use single neurons to control the world today, you use muscles to control the world, then it takes the activity of hundreds, if not millions of neurons up in the forebrain, to coordinate activity of your muscles to move and then say, type on a keyboard. Our approach tries to say, let's not focus on the movement, let's just actually listen to individual neurons down at that muscle interface and see if you can use that to control a machine to otherwise type without actually doing all the movement of typing.
Anthony 09:36
I know that the hand is especially interesting to you. So maybe tell me a little bit more about the hand and why it's a better template for controlling computers than the voice, let's say.
Reardon 09:46
I think voice is a great way to control computers, especially since we use computers as a communications tool all the time. So using it to control communication streams is fantastic. That said, if you think about how you actually control anything in the world today, whether that's sort of driving a car or making a cup of tea, as I just did this morning, it involves the use of your hands. It's not shocking for me to assert that what allows humans to stand out against all of animalia is this ability to use our hands very dexterously. It turns out, if we go back and look at the neurology of hands, you have more neurons in your forebrain dedicated to controlling your hands than any other part of your body. And it's actually kind of by far more neurons are dedicated to that, somewhere estimates around twice as many neurons are dedicated to controlling your hands, as are dedicated to controlling your mouth for speech production and feeding. So it's incredibly densely innervated. The reason it's densely innervated is because you need this adaptable motor skill, this ability to do lots and lots of different things, not just say, walking, you need to do lots and lots of dexterous manipulations with your hands. And you have this amazing adaptive motor skill and inbuilt into your hands, that I always kind of make the joke that your hands make your legs look really, really dumb. And it's really kind of the way it plays out, your legs don't really do much adaptively, but boy, your hands can do a whole lot. So we like to listen to the neural signals that innervate the hands and get you to repurpose that. Because it's, again, I want to emphasize, it's where you have the most adaptive motor skill, not sort of reflexive motor skill, etc, but adaptive motor skill.
Anthony 11:35
I mean, if I recall correctly, for medical school, there's actually only 14 muscles that control the hand. And they're actually all in your forearm, not even in your hand. So is it right to think of this as basically a 14 degree parameter space and we're just always navigating it?
Reardon 11:51
Yeah, boy, this is kind of a deep question. So there's more than 14, but what you identified there was what we call the extrinsic muscles. So most of how you move your hand, if I'm wiggling my fingers right now, is by activating the extrinsic muscles in my forearm. And it's kind of interesting to think that most of the quote, unquote, intelligence, in my hands, is actually embodied in my forearms. There are intrinsic muscles in the head, like the pollux muscle that manipulates your thumb and in many dimensions, but we don't actually listen to those muscles, we only listen to those extrinsic ones that you just talked about. So if we were to reduce this to like a mathematical model, we would say basically, that everything that you do as a manipulation with your hands reduces to those 14 dimensions, you just said. That any one muscle can actually only turn on or off, and it's a degree of how how much on it goes. And therefore that sort of each muscle becomes we think of a plot of an x and y plot. As I activate the muscle, the amount of force goes up and the amount of neural activity into that muscle goes up, we'll call that a single dimension, that as you mentioned, that would seem to say that we can only reduce all of the activity of the hand down to say 14 dimensions. What we've been working on and is really kind of the thrill and the neuroscience discovery for us is what happens if we're actually able to kind of break that model, and allow us to treat each muscle rather than as a kind of single dimensional mathematical object to actually treat it as a multi dimensional object. Can we actually turn the neurons off and on the ones that innervate that muscle in such a manner that you actually like massively increase the dimensionality of an individual muscle? That seems like a mathematically abstract concept. But at the same time, that's what we do in computational neuroscience. We like to make these kinds of like gross simplifications to try to understand the information that's being carried from the brain out to muscles.
Anthony 13:55
Yes. So is it the case that actually, our brains actually work with more than 14 dimensions? Or is it that you're trying to increase the intrinsic dimensionality of the, the hand relative to what Mother Nature has done?
Reardon 14:08
Yeah. Okay. So this is a really good question. What is the dimensionality of the brain that would just say, the motor output system? First, I don't know. But what I would say is one of the things that makes adaptive motor skill so interesting is maybe maybe there's, say 100 million neurons that are part of the motor cortex, the output part of your brain that are trying to control muscles, or maybe say there's 50 to 100 million just at the call at the top end of your brain that are dedicated to turning on and off these muscles in your hands. By the time you get down to the final neurons, these are upper what we call upper motor neurons that exist up in the cortex and then they send down that long synaptic wire down to the lower motor neurons, the ones that exist in your spine. And by the time you get down to that you have this massive decrease in dimensionality from, say 50 to 100 million neurons all the way down to for a single muscle, maybe 1000, maybe only 400, say, the number of neurons that actually directly controlled, the muscle is very, very, very small. They're about four orders of magnitude or more smaller than the number that exist up in cortex that are starting that kind of cascade of signals that actually control the muscles. In and of itself, there's this massive dimensional collapse. And it's, you know, theorized that, the main reason we do that is because control has consequences, that when you turn muscles on and up, when you move, when you are seeking prey or doing anything skillful in the world just to survive, that really, really requires sort of exquisite control. And that dimensional collapse allows you to get increasingly precise control over the, what we call, the end effector. The end effector is your hand, or the end effector could be any part of your body. But in this complex system, it takes a massive amount of computation, 50 to 100 million neurons up in cortex doing their job to get down to that fine control, it's only a couple of 100 neurons, and then ultimately down to just those 14 muscles.
Alex 16:22
So in a sense, by measuring the output at the musculoskeletal level, or maybe at the spinal cord level, but ultimately manifest and how the muscles are moving, you can take advantage of this incredible dimensionality reduction and abstract away a lot of the, I guess, complexities of control that have to do with being a physical being. So it seems like you've kind of hacked into a very high bandwidth highly, I guess, highly processed output modality for the human body. So I'd love for you to tell us more about what you made to do that, what you've developed.
Reardon 16:57
Yeah, I probably have to set up one more mathematical concept here and get to what we've actually been doing with what we call surface electromyography, which is the technology we use to detect these neural signals. People who study neuroscience probably study this the first semester, they took a class in neuroscience, which is work that I think Eccles did in the 1950s. And this is something called motor recruitment. So this is basically when I want to turn a muscle on, I start firing neurons in the spinal cord, these motor neurons, and those cause the muscle to contract, they electrically spike that releases neurotransmitter, the neurotransmitter invades the muscle. Inside the muscle, there's this cascade of signaling via calcium, that causes another electrical pulse inside the muscle. And that causes binding of myosin proteins, and that causes the contraction of the muscle. And then when you want to turn that off, when you want to relax the muscle, you just stop sending that neurotransmitter. And then the muscle goes through a slow relaxation. There's something really important here called recruitment, which is, as I want to increase the amount of contraction I'm doing, I just turn on additional neurons. So let's say there's 100 neurons as a made up example. There's 100 neurons that innervate a muscle and each of them has a name sort of like Alice and Bob and Charlie, in order. When you want to turn on a muscle motor recruitment, this theory, generally accepted in neuroscience, was that you always turn on the same neurons in sequence. So Alice gets turned on first. And then Alex gets very chatty and maximizes the amount of neurotransmitters she's releasing. And as she keeps increasing, she recruits Bob, Bob starts firing and sending neurotransmitter to the muscle and in turn recruits Charlie, and so on. If you follow that through mathematically, what it tells you is that, at least according to Eccles, there's only one dimension of activation that the muscle only gets turned on by the same exact neurons. And then those neurons reduce their activity in a backwards manner so that when up from a measurement perspective, I'm effectively only able to see a single variable, something that goes from, you know, zero to 1000. But it's in the same sequence every time. Our great goal and what we have proven to ourselves again and again and again, is that motor recruitment is not a guarantee. And in fact, there's the bible of neurosciences called Principles of the Neuroscience,
Alex 19:42
The big thick book.
Anthony 19:44 Yeah, every medical student reads that.
Reardon 19:47
It's actually a fantastic book, but it is it's not one that I would just pick up and start reading it is definitely a reference. And in that book, they really emphasize this point about motor recruitment that this is the only way the body works, the body turns muscles off in a single dimensional way. Our simple task, I should say our goal at CTRL-labs was, what happens if that's not true. And we said, well, maybe people just haven't really tried hard enough to look at how you might activate the neurons differentially. So for all the computer scientists out there, if I say that A always comes before B, and B always comes before C, you can see that there's an information collapse such that A predicts B, B predicts C and vice versa.
If instead I said, Alice, this neuron A can fire and then turn off. And then Charlie can start chatting, and then turn off. And then Alice and Charlie can start chatting. And then they can turn off. And then Alice and Bob can start, if I can do that, I now combinatorially massively expand just with three neurons I’ve gone to say eight states of activation. And in fact, there's a rate code in there. So they can fire anywhere from say, one time per second to 50 times per second. And you get something really, really intriguing out of that which is a dimensional expansion, such that out of just a few neurons, active at such a level that you're not even actually moving, generally takes like dozens, if not hundreds of neurons to actually like really move. If I can actually like, learn how to do that, as a person, I now have this ability to actually use these neurons to communicate to a machine without actually even moving or while moving, but without seeming to control the machine, which is one of my favorite gimmicks that we show as a demo is this ability to say, pick up and take a drink of tea, all while I'm still pushing buttons. And then I can put the cup down and now push these virtual buttons, I'm able to actually use this neural code independent of how the neurons are actually used for actual movement. So that's the core of what we've done is set ourselves this goal of overturning one of the accepted laws of neuroscience, this idea of motor recruitment,
Alex 22:09
I love generally the idea of textbooks being not only thick, but wrong. There being a starting place of what we thought used to be true, and then every single piece of what's written is really up for grabs, right, it's up for re-investigation. And I love it when people can build not just a research programme, but a company around that idea. So my understanding of the insights that you're building on is first that the motor neurons in the spinal cord are already very compacted from an informational perspective that the brain is doing a lot of planning, but then ultimately, the control is much, much lower dimensional than what's going on in the brain. But it's not a complete collapse of dimensionality. So each muscle isn't just one dimension, where, you know, there's a dial, which is like off all the way up to one to 10 to 11, of muscular contraction. And the way that that's implemented is by recruiting progressively larger number of neurons in a stereotyped order. But it's actually much more complicated than that, that you can recruit cohorts of neurons, maybe different neurons within a motor pool to drive a single muscle. And then perhaps, if you actually know what individual neurons are doing, you can infer something richer about a person's motor intent than you could if you just recorded the bulk activity of the muscle. Is that on base?
Reardon 23:30
Yeah, I think you're right. So, but I want to be careful with that word, intention. It's a word that means a lot to us that we are translating not your thoughts, but really your intentions, that you have a desire to cause something to happen. And that as you go towards that action, you start to generate these neural signals. And we see that we have an example where we try to get you to push a button before we can tell you that you're trying to push a button. And of course, it's impossible because the actual action of pushing a button happens not sort of neurologically, later. We all accept that. But literally, mechanically later that the electrical pulse that starts to contract a certain set of muscles that would allow you to push say the spacebar on your keyboard happens, say 100 to 140 milliseconds before you have sufficient contraction of the muscle to actually push the button. So clearly, we can decode the motor intent there, a clearly expressed intent that will follow mechanically from your body. But because we can get it and translate that into machines and say, Hey, you're about to push this button, I know you're going to push the button, even if you try to trick me and pull back. I know what that looks like electrically as well. So the nice thing is, you actually when you start repeating that again and again, start to do these actions much much faster, because I'm no longer waiting for my body to go through with pushing the entire button, that if you play that out 100 to 1000 to 10,000 times a day, and think about how you adapt to that as a person, you start to see how you go faster and faster and faster with your interactions with machines, you're no longer waiting for the mechanical act to complete. All you care about as a person is did the machine understand me. And since the machine is just trying to listen to your neurons, yeah, it understands faster than it would mechanically.
Alex 25:27
Tell me how you actually detect this motor intent before it actually occurs.
Reardon 25:33
So we have a device, I'm going to put on a, what's called a 16 channel device right now. And this device is an electromyography device, it's actually really pretty exquisite. I think it detects signal that even a medical device today a $100,000 medical device could not detect. And basically, it's 16 channels that I lay around the wrist, and it detects differentially, so it detects an electrical wave as it passes as your muscle responds to neural input. And let me, let me, I'm going to hold up this bracelet here, and maybe you can explain what people see.
Anthony 26:11
Yeah, sure. So what I see is, looks like a normal wrist fan. But on the inside, it has, I don't know, 20/30/40 little brass knobs that look like there are sensors that would pick up electrical signals. And then you basically put it on your wrist just like a normal wrist guard. Yeah, it's kind of a sweatband for the brain.
Reardon 26:30
Yeah, perfect. Actually, it's very funny. You said that our very, very first demos, it's named after avenues in Brooklyn. And I cannot remember what the A, was Bergen B, Bergen was actually a sweatband that we sewed electrodes into.
Alex 26:48
And just to check really quickly, that thing is wirelessly connected to a computer, right? So do you have to be tethered to a phone, or…
Reardon 26:56
It's pretty important that it be wireless, it actually just speaks Bluetooth, what happens is a motor neuron in your spinal cord spikes. And just to get this across to folks who have no neuroscience background, a spike is an action potential. It's quasi digital. So basically, an electrical wave is generated by a neuron or by your muscle fibers. And that electrical wave is the exact same every single time. So we see this little voltage deflection inside of the muscle fiber or inside the neuron. So this band sees that little electrical pulse as it goes down the muscle fiber, that electrical pulse as it's going down, the fiber is causing the contraction of the muscle. And this happens trillions of times per day across your entire body. So we see that inside the device, and then we start to decompose the activity of lots of those little pulses together, we decode that into the component signals of individual motor units or individual neurons,
Anthony 27:59
How long does it take people to learn how to control the computer with this device?
Reardon 28:03
For most people, it doesn't take anything. Because how we start is we say, well do something natural, we're going to understand you by you moving naturally. And then over time, I'm going to say you relax into neurological control from physical control, or what we call myo-control, meaning muscle control. Something that we've showed many times is this ability to like, just pinch, I'm just going to put my thumb and index finger together and pinch, and I might hold it and then release it. And by doing that, I've got what I called the perfect button. It's just always available, it's on my body. And I just want to be able to push this button, hold it, release this button, you can use it for lots of different things. And I do that by literally just articulating, by moving visually moving my thumb and index finger together. Now, what ends up happening is you effectively get into this kind of conversation with the machine where rather than doing a full punch and release, as we call it over time, you just do smaller, smaller ones. And by overtime, I mean minutes. And then in the end, I'm doing a pinch release now and I'm not articulating my finger at all. Instead, my fingers are just kind of pulsing and the muscles are pulsing without me articulating. And at a certain point, I can no longer feel it. That the machine has learned that Oh, you're only activating a couple neurons and a couple of opposing muscles. And that's sufficient for me, machine to understand that you're trying to push a button right now. So this idea of learning how to do these interfaces is all based on something we call co-adaptation, which is a machine and you adapting your understanding of a neural code in real time. In some sense doing it ad infinitum. You never stop doing it. You're just always changing slowly. how you actually activate something.
Alex 30:01
So for a mechanical task like typing, I don't have to improve my skills, the computer will just adapt to me.
Reardon 30:09
That's absolutely right. That's that's exactly the dream here is a machine learning you rather than you learning the machine, go. And by the way, this is how you work today, when I do something that is maybe the most skillful thing I do in the world today, which is like take a sip of tea, I'm going to raise the glass up to my lips and tilt it back and pick a small sip of tea and put it back down. I've just done something incredibly computationally complex, robots are terrible at this today. But for you as a person, you do it skillfully, every time, even though the task has changed. When I picked up the glass, I didn't shove it through my face, I adapted to the weight of the glass, I adapted to the texture, adapted to how much liquid was in there, and I tilted it back. And I slowly drained the fluid into my mouth, I didn't spill it anywhere, I did it all very, very skillfully. And each time I take a sip, the task changes, there's less fluid in there, it might be a little bit colder, etc. It's always changing, and you're always adapting to it. And in fact, you do all of this without even thinking, I just took a sip of tea, I didn't think, Oh, I've got 27 muscles in my arm, how do I alternate and turn them on and off, et cetera, I don't do that I just take a sip of tea. So similarly, you just push a button. And I don't train you to activate a certain muscle, you just push a button. And because you slowly do less and less to do that, which by the way your nervous system does automatically, you're always trying to minimize the amount of effort it takes to actually achieve some end effector goal, some end goal, you can't stop doing that. It's just something your nervous system is wired to do is minimize effort like that. You just relax into control with this machine. What we think of is reducing the error manifold between a human and a machine, such that you come to an agreement on the minimum amount of neural activity to achieve a goal.
Alex 32:06
It’s one of these paradox The idea of Moravec’s Paradox, which is that things that are easy for us are insanely difficult for computers and things that are insanely difficult for us like adding very, very large numbers can be done trivially by computers by virtue of just how much time evolution has spent making things easy for us, right. So you say it's easy to take a drink of a cup of tea. But you have to decode those signals in a way that evolution has designed very carefully to produce. So tell me a bit about the machine learning and the statistical approach to translating these electrical impulses picked up via electromyography into a representation of what's actually being done by the person.
Reardon 32:47
Yeah, really good question. So what I would say is we use techniques from the spread of contemporary machine learning. We started out, for instance, working on problems of representation. And anybody who works in AI or ML will know like, a lot of the breakthroughs over the last decade plus in applied ML have come in, what I'll call areas of representation. From there, we use techniques from reinforcement learning any of the recurrent network techniques you might have heard of from LSTMs on. And of course, now we're exhaustively using transformers in different ways in our stack, it is not a single one size fits all ML approach, it is kind of mixing and matching different ML. Now one last thing I should point out here is most people who work in ML, and especially in my company, work on models that are massive, like multi billion multi trillion parameter models. And those things are sort of learned and then model compiled and model issued. In our world, we're working on that problem of real time model adaptation. So how does the model itself continuously adapt in real time? And I consider that to be kind of one of the most exciting and kind of most, at least currently under explored areas of ML.
Alex 34:08
So thinking about the future, then, I mean, you've, you've perhaps satisfied your curiosity, or are satisfying your curiosity around motor control. But where are you being taken next?
Reardon 34:21
What I've said recently is I finally found something I think I could work on for the rest of my life, because there's so many questions open. I like following where my ignorance inspires me. And I can honestly tell you, I didn't feel that way about the web at all, even though I felt like I, you know, had my hands in helping form the web, the first web in the 90s. I didn't feel like there were problems to solve till the end of time. I thought most of the problems were going to be ones that we created ourselves, and that we would try to, you know, unwind those problems over time. And frankly, I don't think I was far off the mark.
When it comes to neuroscience, there's just so much more to learn. And there's so much more to learn even outside of academia, if we have these approaches that try to think about things from a kind of augmentation perspective, rather than a clinical or pathological perspective, that there's so much more we can bring to people to help them understand this incredible tool, this incredible thing that we are. I am somebody who thinks we are our brains. And I just can't, I can't imagine ever getting bored of that topic.
Anthony 35:29
This is an amazing conversation today. Tom, thank you so much for having it with us.
Reardon 35:33
Great. Thanks for having me, guys.
Alex 35:35
It's been a pleasure. Thanks for your time.
Anthony 35:45
Let's move on to the Hammer and Nails part of our podcast where you and I talk about nail, a problem or a hammer, the solution in honor of our in person meetups in Boston many moons ago. Alex, what has this episode inspired you to think about today?
Alex 36:00
I think it's a hammer. But first I do want to like just harken back to those meetups just for like 30 seconds, they were really special. This notion where you're getting a bunch of people together to talk about new solutions, new technologies that maybe people haven't heard of before. And new problems that people might not think are tractable. And just actually hashing it out together and sharing that was really, really special. And it's something that I'm thinking of bringing to the organizations that I'm a part of just maybe as a lunch event or an after work event just to get people to mix problems together. So I'm glad that we're doing this at the scale of a podcast now. But sometimes I kind of think back to the olden days. And those were really special, special meetups.
But today I have a hammer, I think I want to turn back to computers giving us input. And what Reardon talked about is us giving input to computers. But the core of what we're talking about here, in this series, we're talking about giving computers human abilities. And one subset of that is giving computers human senses. And there's three parts to doing that for any sense, giving the computer the ability to read part of the sensory world. And that's what Reardon is doing with CTRL-labs and now at Meta with his bracelet is reading the electrical activity of muscles and translating that into information that computer can use in order to allow a tighter link, there's reading, then there's mapping, understanding processing, what's out there in the world, organizing it allowing you to manipulate it. So for vision that would be Photoshop or the JPEG compression algorithm. And then there's writing, there's taking a representation in the computer and putting it back into the real world. Right?
So I want to talk about is haptics in its broad sense, which is any system that incorporates tactile feedback, so writing back into our human sense of touch, and they can do that through all kinds of different mechanisms tightening around the skin vibrating. I'm interested in how humans perceive touch. I think there's a lot that's been discovered about touch that's truly extraordinary, that hasn't made it into the world at large. Because one thing that I believe folks think is human touch is just one thing. Whenever you touch a piece of your skin, there's one number which is like “How hard are you being touched”, and that number is varying over time. And that you have a body that's covered in pixels that's constantly reporting, you know how hard any part of your skin is being touched. And that couldn't be further from the truth, as is the case with biology, there's always more complexity lurking under the surface. And I just want to, I want to give a sense of what's been learned here. And one of my I, consider mentors or people intellectually that I look up to in the world of touch is David Ginty, who's a professor at Harvard Medical School. And he was there while I was training to study this sense of smell, and I learned a lot about touch from him, and also from the papers that he's written. So I just want to give a little whirlwind tour of, of just like what touch is, and how it's actually much more rich, its higher dimensional than just how hard am I being touched to this particular pixel of skin.
So the first thing I think to understand is, there's different kinds of skin. If you look at your hand, there's likely going to be more hair on the back of your hand or on your forearm, than if you turn your arm over and you look at the palm of your hand or the forearm that's closest to your elbow. And those are two different kinds of skin. So the kinds of skin with hair is called hairy surprise. And the kind of skin that has no hair is called glabrous G L A B R O U S, impress your friends when you're out to drinks with your glabrous skin knowledge.
The information that you get from these different parts of skin is different. So there's different cell types. If you look at your hand, in just a patch of skin the size of a dime, there are hundreds of 1000s or millions of sensors, that all sense different things. So when you stub your toe, for instance, there's the recognition that your foot has stopped and that you've run into something. And then a moment later, there's a rush of pain. And that's because there's two different types of information that are making their way to your spinal cord in your brain at different rates. There are pain fibers that are open nerve endings that sense pain, but they transmit information very slowly. Look at all the different kinds of things you can sense. So when you put your finger on your palm, there's a type of neuron that can sense, light touch, just small indentations. And some of those cells are called Merkel cells. Some of those are called Meissner corpuscles. There's all kinds of different funny named cells that are responsible for this. But let's say you start to push harder, different types of cells become activated. Let's say instead of using the flat of your finger, you use your fingernail, that sharp indentation, and there's different types of cells that sense that eventually it turns into pain. And then pain fibers start to activate. Right, so we're already talking about, you know, a half dozen different cell types. Now, let's say you put the flat of your finger on your palm, and you move your fingers slightly and stretch the skin, there are different cells that detect stretch, the deflection from your skin away from its normal position with respect to the bone. That's how you kind of know if your fingers are bent and things like that, that's one of the signals that your brain gets.
And then if you turn your hand over, if you've got hair, if you lightly deflect the hair, there are cells that detect deflection of hair, there are cells that detect the soft stroking of skin, there are different cells that detect the pulling of hair. And some of these cells have names like, longitudinal lanceolate ending, or circumferential ending or Pacinian corpuscle, and some of them just have generic names like free nerve ending, and we don't necessarily fully understand how all of these senses and all of these sensations are actually biomechanically gathered from the world and how that they're transferred to the spinal cord into the brain and processed. But there's been decades and decades of work. And I think the takeaway is, is that first of all, you're a miracle, right? You're covered in one of the most advanced sensors that we know of. And it's watertight, and it's largely impact resistance. And it can bring you great pleasure, and it also can bring you great pain. But those sensations are constructed from sensors that are embedded into the watertight layer that is your skin. And I just for me, at least every time I reflect on our human senses, and I really get into the details, the closer you examine how we work and how our senses work, the more miraculous it seems, the more improbable it seems. And the more wonder, and curiosity, I feel. So I just wanted to give a little bit of a taste inspired by some of my mentors in neuroscience and just say, like, look, whenever you talk about a human sense, it could be touch, it could be sight, it could be smell, which is my favorite. There's a world of complexity, a world of richness that's inside things that we think might just be one thing. And by looking very closely, you can fall into this world of possibility. And I think that's just endlessly rewarding.
Anthony 43:22
Amazing. That was inspiring Alex, it's always such a pleasure to hear you talk about this. And, you know, you made me wish I had been a neuroscientist.
Alex 43:29
You too there’s time. I know Reardon changed his whole career and went back to school, became a neuroscientist and then uses neuroscience in a company. So if he can do it, anybody can do it. It's possible.
Anthony 43:42
All right, you know,
Alex 43:42
there's the heart and the mind, maybe I'll start the next career, the mind instead of the heart. But in general, I think we're a really good pair with the heart and the mind. So if you want to leave the neuroscience to me, I'll leave the cardiology to you and will continue hand in hand on to the future.
Anthony 43:57
You know, it's funny, as we're closing out here, when I was in high school, I played in a band, which was 90s era indie rock, and it had a very pretentious name, which was Eros and Psyche, you know, mind and heart. So, so yeah, we can call ourselves Eros and Psyche.
Alex 44:12
Okay, that can be our April Fool's episode. Welcome to Eros and psyche. Exactly. All right, Anthony. One thing that this all makes me wonder after having talked to Reardon about reading muscular activity, is what we're going to learn in the next episode about controlling surgical robots. Right. So this very much spans the read and map and right architecture of digitizing a sense. If we can read what a person is trying to do with their muscles can be righted out into the actual actions of a robot. And one of the most sensitive applications of a human moving their body is in surgery. So what I'll be curious to learn is, is there a future where we're actually reading directly from them? sort of surgeons, basically from their minds almost with just a few synapses difference in translating that into a robot maybe in some other room, maybe in another part of the world, doing life saving work to surgically help someone. So we'll have more to investigate there, but I'm very excited for that conversation.
Anthony 45:19
Me too, can't wait. Great talking to you today.
Our thanks to Dr. Thomas Reardon for joining us this week. Next week, we will be exploring the world of surgical robots. We'd love to know what you think. Write to us at theoryandpractice@gmail.com or tweet @GVTeam.
This is a GV podcast and a Blanchard House production.
Our science producer was Hilary Guite.
Executive producer Duncan Barber with music by DALO.
I'm Anthony Philippakis.
Alex: 45:49
I'm Alex Wiltschko.
Anthony: 45:51
And this is Theory and Practice.