S4E8: Dave Munichiello on Investing in AI’s Future
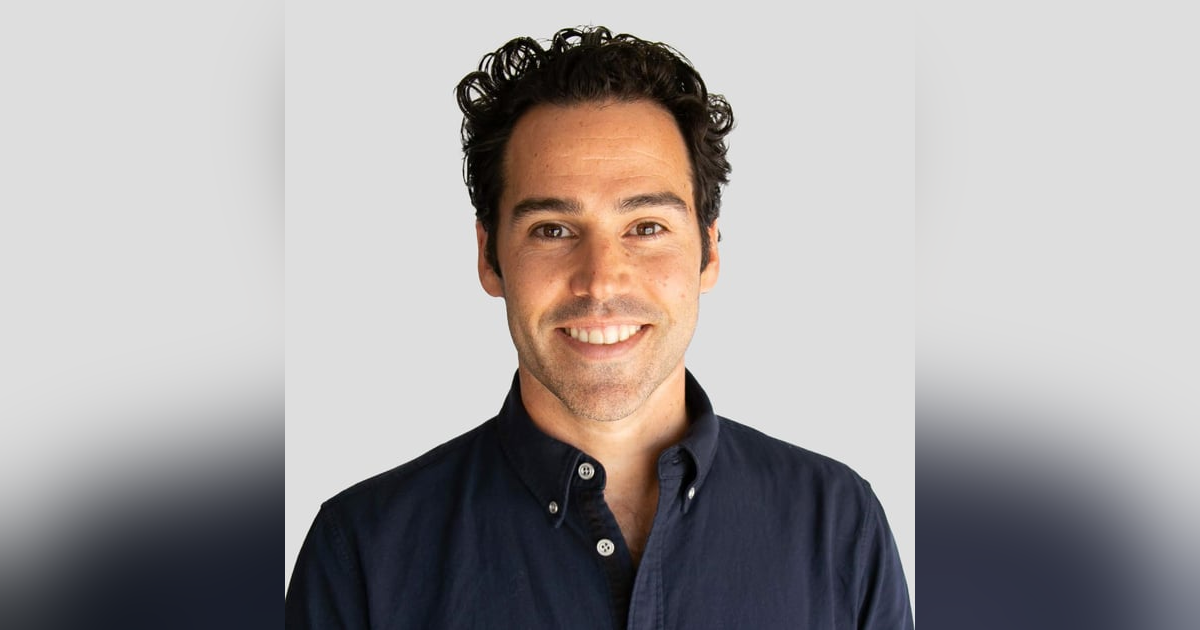
In the final episode, we reflect on investing in artificial intelligence's future with the leader of GV’s Digital Investing Team, Dave Munichiello, who has a long-standing history with AI and robotics.
Throughout the fourth season of Theory and Practice, we explored emerging human-like artificial intelligence and robots. We asked if we could learn as much about ourselves as we do about the machines we use. The series has covered safety guardrails for AI, empathic AI communication, communication between minds and machines, robotic surgery, computers that smell, and using AI to understand human vision. The most recent episode with Google DeepMind's Dr. Clément Farabet illuminates how computers might demonstrate understanding and reasoning on par with humans.
In the final episode, we reflect on investing in artificial intelligence's future with the leader of GV’s Digital Investing Team, Dave Munichiello, who has a long-standing history with AI and robotics. Dave was an early technologist at Kiva Systems, purchased by Amazon and ultimately becoming Amazon Robotics. Over the past decade-plus at GV, Dave has been leading investments across two major categories: Platforms Empowering Developers (GitLab, Segment, Slack, RedPanda, etc) and Platforms Powering AI Systems (Determined, Modular, SambaNova, Snorkel AI, etc), along with others. Dave’s first AI investment, Lattice (bought by Apple’s Siri team) was seven years before the hype of generative AI. We asked, from a seasoned AI investor's perspective, where does AI hold the most promise?
To answer this, Dave returns to the themes we've investigated over the last eight weeks — including AI trust and safety, which Google Health's Greg Corrado raised in the first episode. Together, we explore how AI will change how we work, the nature of jobs, and how an investing team with a culture focused on having more questions than answers is well positioned for AI’s future.
Dave rounds out the discussion with a picture of how artificial intelligence, with real-life use cases, will move research lab theory to real-world practice. He also walks us through his hopes for AI, including a world where humans and computers exist as co-pilots.
Ultimately, Dave shares an optimistic and rational view of AI's future. “AI has the potential to democratize the very creation of technology," he reflects. "With AI-assistance, folks across the country will no longer need to rely on software programmers to solve everyday digital problems – they’ll be able to create these tools themselves. That is incredibly exciting, and I'm honored to be a part of that journey."
Anthony 00:00
Hello, welcome to GV Theory and Practice. This is the last episode in our series exploring what it means to be human in the age of human-like AI.
I'm Anthony Philippakis.
Alex 00:11
And I'm Alex Wiltschko.
Anthony 00:12
Today we are going to bring our series on learning about ourselves as humans from understanding AI and robotics to a close,
Alex 00:18
We'll be drawing out what we've learned from our wonderful guests this series.
Anthony 00:23
We're going to add a fresh perspective as well. And look at what makes human-like AI investable. So who better to join us in this conversation today than Dave Munichiello GV General Partner, and leader of the Digital Investing Team. He has a wealth of experience investing in companies using AI and robotics. And he's looking ahead of the curve. Dave, welcome to theory and practice.
Dave 00:46
Hey, guys, thanks so much for having me.
Anthony 00:48
So, you know, to kick things off, you've had a long standing history in both AI and robotics. Maybe you can tell us what you've learned and how you got started.
Dave 00:56
My background in robotics started with a company called Kiva systems. So out of graduate school, I met the founder of Kiva systems robotics company. The founder, Mick, had a big idea. So he was at a company that did grocery delivery before grocery delivery was cool. And that company was losing a tremendous amount of money on every single order that went out the door. And he wanted to find a way to run operations in a way that didn't lose a tremendous amount of money every time every order went out the door. And so he started looking for a solution to that problem, he started looking for a tool that could help him build a company that would enable the world of e-commerce to deliver goods very, very quickly. And so he created the company Kiva systems, and I always describe the company as reluctant roboticists, we were not roboticists that were excited about robots that wanted to build them from day one. We stumbled into robots as the only viable solution to solve this tech problem and ended up building out a really interesting robotic system.
Alex 01:59
So your solution was to get the, I quote, ..”he shelves to come to you”. You had robots respond to customer requests, and bring the requisite items to human packers massively increasing productivity.
Dave 02:10
Yeah, so Kiva grew to be, you know, $120/130 million in revenue and sold to Amazon for almost $800 million. which back then was a big exit. I came to GV, after my time at Kiva. For the last 10 years at GV, I've been investing in software companies.
Alex 03:13
Building on that, this idea of investing in companies that are adding value right to either to an ecosystem or directly to a consumer, this new breed of machine learning models, in some ways is a continuous improvement along an axis that we've seen before. But in many ways, it is qualitatively different. It really feels like these systems can do things that the previous systems couldn't. And I don't know if that's a feeling or not, but in terms of thinking about these new human-like AI systems, what we have in the modern day, right? How do you view how they add value to companies and to people with respect to the systems that came before, particularly in healthcare.
Dave 04:01
I think what we're seeing in the market is a set of tools and solutions that increasingly feel more natural to interact with. And that builds trust. And, you know, we saw it at Kiva, we tried to make the robots look as human as possible to help users trust them. At GV, we use AI to help our investors and building trust between the folks who were building that AI. And the investors who are out there using that AI is incredibly important. And I think I can imagine that doctors, patients and researchers interacting with AI are finding it to be more human, more intuitive, easier to work with. And as a result that will build trust over time, that these systems will be more helpful and that the direction, the orientation of where things are headed is towards a more promising future and not a future of frustrating tech, that could be complicated to work with.
Alex 04:54
It's interesting that you mentioned trust here. That was something that Greg Corrado told us about in our opening episode about safety when he was introducing these systems. And he said something interesting. In healthcare, you can only advance at the speed of trust. So with all these developments of AI models, machine learning models have really taken a step change in terms of their predictive ability in terms of qualitatively what we think that they can do. It seems like there's an early demonstration of understanding or at least a statistical grasp of large swathes of language and meaning. How does that change things? From your view? What's new here?
Dave 05:38
Yeah, I love the framing a statistical analysis of a large swath of language, because I don't think that we're at a place where we're seeing intelligence, I think we're seeing, you know, computers start to understand how humans communicate, and start to understand how language patterns are formed, and systems are able to do massive amounts of retrieval, and prediction of what these patterns of communication could look like. And I think that will improve over time. And the things that we get really excited about are these opportunities for zero to one improvements in the world with new technology, and opportunities for these virtuous cycle platforms to be created. We're not looking for point solutions. We think that AI is on a trend right now towards being more interesting, more useful, more successful at creating value for customers and companies and users over time. But we're not quite at the inflection point where we're just spawning new businesses. We tend not to be hype driven. We didn't jump on the crypto train aggressively, you know, we, sort of like, dipped our toe in the water and experimented and learned a little bit. And I think we view generative AI, similarly, interesting capability, lots of fun stuff happening. Like the crypto trend, there's a little bit of, you know, the world of tech sort of sees this as potentially the next platform with a lot of people sort of placing their bets and trying to claim that there are generative AI company, and they were a crypto company like, like a year ago.
Alex 07:09
I guess I wanted to ask how investing is working in this new age of human-like AI? Because what I'm hearing from you is, well, let's wait and see. We don't know, like what's going to turn out here. But ultimately, the fundamentals come down to what's the value that's being brought to the consumer. And it sounds like you're looking at things through the lens you're looking at before and resisting, being pulled into this being like an absolute sea change. Right. But it sounds like you're looking for value opportunities here.
Dave 07:36
So I would say that we're investing hundreds of millions of dollars in companies driven by AI. We're doing it at many different levels of the stack. But we haven't come out with a here's where we see the future going. Because I think right now we have more questions than answers. And I think that's generally true of folks who are deeply curious and trying to learn as much as they can about a new space.
Alex 07:59
We're capping off our series about what's different in the world now that AI is increasingly human-like, and I want to, I guess, go a couple clicks deeper into a specific area, and just kind of return to that theme, which is, if we're talking about capabilities and technologies that are adding value to people through the vehicle of a firm of a company, tell me about how you see this playing out in companies that are working in the healthcare space.
Dave 08:34
I think anytime there are large amounts of data that are untouched, there are interesting opportunities. So in the AI world, we've invested, you know, as low as chips, and compilers, and programming languages and data platforms. At every layer of that stack. There are interesting new innovations happening. What does that mean for the implications for the market? Compute becomes cheaper and cheaper and cheaper over time, almost indefinitely, as far as we can see. Capabilities of compute become, you know, nearly infinite over time. We can imagine just having vast amounts of compute vast amounts of resources, and what can you do with that? So we have customers that are saying, it's awesome to be able to throw a bunch of text into ChatGPT and get an answer. I want to throw the whole human genome into something like ChatGPT. And I want to start to see patterns in that. Can you do that for me? I want to throw weather patterns. Yeah, I want to throw the most complex data that we have in the world into a system. And I want to start to see forecasts, I want to start to see predictions of that data. And, you know, the human brain can think of 30 or 40. Other ways that we can take things that we don't understand. And actually the human brain is something that we would love to understand better and is quite complex. How do we, how do we gather as much data as possible from as many sources and start using AI to predict where things are going, that's interesting. And so I think that applying to healthcare, you gather a bunch of data about a human, you could have machines start to generate hypotheses about what's going on with that human. And you could give the doctor, the human doctor, an immense amount of leverage, they could see many times more patients, they could engage in totally different ways, they could focus on the human side of interacting with patients. Quite interesting.
Anthony 10:42
So Dave, I know you've been listening to our series. What's hit you?
Dave 10:50
I mean, I loved your dive into all of these sensors connected to the human body, right? Like, this is just fascinating. I think, you know, the Moravec paradox piece was very interesting, this idea that, you know, it's actually quite more complex, to create the human experience in a body, and understand the human experience in the body and move. And create all of that sensor motor and perception skills like that's quite difficult to do. And so it's unlikely that very soon we'll have humanoid robots roaming the Earth, like, I think that's going to take a while, you guys would know better than me in the life sciences side. But what we are seeing is like text, and interaction over text and the way that humans interact over text or voice, with systems, that is going to become very hard to differentiate between what is a human, and what is a machine. And the wonderful thing about machines is they can work 24 hours a day, every shift, you can imagine, you can generate billions of them. And you can create massive amounts of leverage in the world.
Alex 11:50
And what does that mean for the companies that people are starting and the companies that you're excited to invest in?
Dave 11:55
Well, now with generative AI, there are many functions within an organization that either don't need to exist anymore, or it can be just one person. And that one person can be much more flexible, they can cover a lot more ground. And so, that kind of leverage is really exciting for us. So it means that, you know, we have seed companies that are creating whole recruiting firms that are competing with massive well known recruiting firms using just AI. So AI sending an email interacting with somebody generating a lead, convincing them that there's an interesting match, and a human doesn't get involved until the final curation of that initial intro, that's kind of interesting, a bunch of these things scale. And many of them could potentially replace whole industries.
Anthony 13:36
Let's move on to the next phase of our podcast, where we normally shift to Hammer and Nails are a section where Alex and I bring up either a hammer, which is to say, a tool, or a nail, which is to say, a problem to solve. But I think today, since it's a wrap up episode, we have Dave here, we're going to change things up a little bit. Dave, would you be up for joining us to work through the big questions?
Dave 14:01
Sure. I’d love that. Thank you so much for inviting me.
Alex 14:03
So here's the kind of big ideas that have been on our plate. How will human-like AI influence us and change us? How will it compete with us? And I think most importantly, what I'm most excited about, how will it enhance us?
Anthony 14:16
I guess these are the nails that we set for ourselves at the beginning of this podcast series. So maybe we'll start off with that last one to enhance us. You know, I want to kind of talk about the dominant narrative that people are talking about with AI right now, which is the co-pilot. And it's this sort of thing that we will be working with and it will be enhancing us. And a lot of the first generation of products really are kind of leaning into that, Microsoft but much beyond Microsoft. Do you agree with that metaphor? Do you think that's the way it's gonna play out?
Dave 14:49
We are starting to see human-plus AI, which is quite interesting. And I think sometimes we're biased to think that we won't be replaced by AI in many of our jobs. When I was listening to the DaVinci episode, I was listening to the capabilities today requiring humans in the room, in with the patient. And then that one example of when an artery is cut, or when a vein is cut, it starts to bleed. And sometimes it's really hard to find where that bleed is coming from. But if you could just cauterize that bleed immediately, it would be so much easier. And that's really hard to do as a human to reach in and find among all that blood exactly where the bleeding is coming from. That seems like a really solvable problem with a robot. I feel like over time, we will see that AI can be more effective than humans at many jobs. And that is a little scary. And I think it will change the way that humans do the jobs that they do. And I think it could create this cycle of sort of a threat to human roles, and elevation of how humans interact, right, so maybe not human scale in a different way. And eventually, what I would love to see is humans become the co-pilot, AI is doing jobs at all points in time, and humans are sort of overseeing.
Alex 16:01
And that's exactly an idea that Clément Farabet brought up when we talk with him about how he sees this playing out - a surgeon, the job definition of a surgeon changes, right, instead of a person who is actively managing the manipulation of tools in order to make changes to somebody's body. A surgeon is somebody that is actively managing systems that are doing that work that they have done in the past. So one surgeon managing a fleet of robotic systems that are performing many surgeries at once,
Dave 16:34
In so many organizations, there are different levels of say, programmer developer, but they have groups of people under them that are doing different subsets of work, I just think that we'll start to see a lot of that start to get done by AI so that everyone starts to be focused on coordinating a bunch of AI. And that coordination stuff will be where the most interesting jobs are, in my mind.
Anthony 16:59
You know, one of the things that I think it's also interesting to think about going back to the robotic surgeon example, is not just how AI will enhance this, but how it will change us.
So to kind of go sort of nerd out for a second on medicine, there's a part of your brain called the homunculus, which is a mapping of all of your sensation onto your brain. So you can find like a part of your physical brain that corresponds to your arm or your hand or your leg. And we know plastic in nature. And so it's kind of interesting to imagine that, you know, the future surgeon might actually have an entirely different set of motor skills that they end up learning, because of the technologies that they're exposed to. You know, beyond this very sort of focused example, in medicine, I know if both of you have ideas on areas where AI will change us.
Alex 17:57
One area that pops into mind for me that I've been thinking about is along the lines of what Dave has been saying about how organizations might change. So let's think about what the telegraph and what email did to organizations. Those are super mundane things right now. But what they did is they changed a parameter of a really critical property of how an organization functions by orders of magnitude. And that's how long does it take for a message to get from A to B?
that different people in different jobs, the relative sizes of their homunculus change. And so for example, gamers often have a bigger part of their brain devoted to their thumb. And so it's And there's this other parameter that's been largely untouched, which is, how compressed can information get as a function of work, right. So like, you've got a division deep in your company, and they're writing a report, and that gets compressed to an executive summary. And that gets, you know, sent up the chain. And eventually, a decision is made based on an incredible amount of information compression that starts with like raw sensor data or raw like observations on the line. The amount of compression and amount of time it takes to compress things, in order to be ready for a decision is enormous. That really hasn't been touched, right? Except for the latency of like actual physical messages or electronic messages being transformed. But what I'm seeing human-like AI and large language models getting really good at is summarizing things and compressing information.
And so you know, those are the two important pieces of the revolution of how information is being transferred around the world. It's like, what's the bandwidth? What's the latency? And compression has a really critical role to play here. And so if you can compress information throughout your organization, that means you can understand a much larger organization much better, right? And that might mean that you either have smaller organizations that do the work of much larger organizations, or the max size of a company can grow by a huge amount. I mean, the biggest companies that existed 100 years ago, were not as big as the biggest companies today. Right? So that could change as information compression gets better and more accessible.
Dave 19:53
I think in businesses, we're starting to see massive specialization. I sat on a plane two days ago with a surgeon from UCSF, and she was, you know, on her email the entire time on that plane, sending dozens and dozens and dozens of you know, her email box was even more painful than my email box. And I was sitting there thinking she's this world renowned orthopedic surgeon, very impressive human. And she's not in an operating room right now overseeing surgery, she's not doing the most highly valued thing that she could possibly do. She's doing email. And so should she be doing email? Or would AI free her up to spend 100% of her time doing the thing that she's best at? And then I think one step further is, if you're not one of the two best people in your organization at something, why are you doing it? Could those two people be doing the work of everybody in the organization at that sort of professional, superior level?
Alex 21:11
So let's double click on that, right, because that's the flip side of the coin of AI helping us to become better, versus AI competing with us. And what you're talking about is kind of a race to the top, or the cream of the crop, whoever's the best at a thing should do that thing, and just be leveraged like crazy. And then the folks that don't make the cut, or you know, maybe you should be doing something else, they're effectively being out competed, in that specific role by artificial intelligence. So roll that out for me, you know, how, what's the endgame here? How does this work?
Dave 21:41
So I push on that a little bit, I think that what we're doing is we're handing capabilities to organizations so that their two best people can be as good as everybody else's two best people. So you may need fewer people, but the sort of level of performance becomes spread across the very top, you're, you're spreading best practices across the whole ecosystem of enterprises, which becomes quite interesting. I think, in physics is this idea of entropy, you have to add energy into a system in order to drive order, and you guys are closer to neurology than I am. But my understanding is that in the brain, as you practice something over and over and over again, the brain actually has this bias to build a pathway, right, and that drives efficiency. So the brain over time, as you're doing something over and over and over again, builds the most efficient pathway. I think computers will get to the point where they have this intuition, where they have captured the very best in us, and the very best of our subject matter experts. And they can now when paired with an average human, somebody not have exceptional performance, can help them perform like the very best,
Anthony 22:46
You know, that reminds me of the fascinating discussion we had with Claire Cui in episode two, about the future of AI being modular, just like the human brain. So the intuition, which is what we humans do naturally, we pick the right tool, or the right module in our brains for the job.
Alex 23:04
Absolutely. So we're coming to the end of this podcast and this whole podcast series. And I have found it to be incredibly fascinating. And this is the stuff that I love to think about. And I've loved hearing from all the people that have come so deep in different aspects, dragging the future into the present. So let's just spend a couple of minutes thinking about our big thesis for the series that we're going to learn more about ourselves, than about AI by studying it closely. So let me start with Anthony, what did you come away with in the conversations from this series?
Anthony 23:42
I think there were three things that I came away from as recurring themes as we talk to our guests this series. So the first was that at some point, Alex, you talked about Hugo La Rochelle's concept of the receding horizon of human intelligence. And, you know, just, if we look at the history of work in artificial intelligence, people thought at first if you get a computer to do math, that would be intelligence, and obviously - got a computer to do math really early on. Then same thing with chess, you know, if you could play chess, then it would be intelligent, not there. Now, we're looking at literally computers that can write full texts that are indistinguishable from a human. And yet, they don't somehow seem to be intelligent yet. And it's getting harder and harder for us to really nail down what are the intellectual capabilities that make us uniquely human? So I think that's the first thing.
Second thing that I think about is, I think there's something really interesting on this Moravec paradox, that a lot of the things that we think are the hardest to do and that are most uniquely human, actually might turn out to be the easiest for computer to imitate because evolutions had so little time to refine those. Whereas things like motor coordination might actually be one of the hardest things to get a computer to do, because it's been so well sculpted by evolution for billions of years. So I think that's another thing is what's hard and is easy, is not at all clear and a little bit surprising.
And then the third thing that my brain goes to is I still, and I've touched on this a lot, and maybe it's my own weird kind of obsession. But this interplay of induction and deduction, where the first generation of artificial intelligence, good old fashioned AI, GOFAI, was all rules and deduction, and it was way too brittle and didn't work at all. And then we've had this amazing 30 year run of inductive systems that are statistical in nature. And yet, you know, every time we make a plan as a human, we're doing deduction, and somehow getting models in place that can flip back and forth between the two, again, I think that's something that still is a hard nut to crack. And, and we're still working on it. So I don't know, these are my thoughts. Dave and Alex, what about you?
Alex 26:10
You know, in terms of the central question for us about whether we learn more about ourselves than we learn about machines, when we look deeply at AI, I learned a lot from Jim DiCarlo. He's a hero of mine. Because he's been doing that explicitly. He's been making machines for the purpose of understanding human vision. And he was very clear that biomimicry as such, has limits. And he says, We love our beautiful ideas. We love our beautiful models that show us that our brains were the best model for AI. But he says, we have to do the work, and make sure that our models fit with the data. I guess a couple of themes have emerged that have solidified in my mind.
The first is this notion of a single system that does it all. So artificial general intelligence being a monolithic thing, the closer that we look into every little success that we've had, and some are mind blowing successes in terms of what AI can do, they're all systems, they're all made of modules, right. And then those modules can be composed together. So the idea of monolithic AGI, to me that idea has receded a little bit.
The other thing is there's got to be ergonomics to this, right. I mean, what I think was so compelling about ChatGPT, when it came out, is the ergonomics are language. And there's almost certainly been systems that are in some measure of predictive power or state of the artness have matched ChatGPT in some way or the other except the interface isn’t language, right? So there being ergonomics that allow people to hook into these things is just really critical. And there's aspects of trust that have to do with that, there's aspects of user experience that have to do with that. I think that that's really interesting.
And then, underpinning all of this, the thing that I find to be frustrating to be exciting to be, I don't know, eye opening is, we are bumping up to the limits of our own minds, right, we're making systems that in some small ways are exceeding what our minds are capable of doing. And yet, we are still the engineers of these systems. And this to me is unprecedented. I mean, like, 2 or 300 years ago, there, there were no systems in the world that could lift or push, or pull or twist or smash, anything more than muscles could. And there were stronger and weaker, you know, animals and beasts on the world. But like there was nothing that was stronger than something living. That era changed. And how society was organized, fundamentally changed once we had systems that were stronger than life. And we're seeing that on the intellectual axis right now. And I have no idea how this is going to play out. But what's really interesting is we still get to engineer these systems, and we still get to point them in areas that make our lives better. Right, but how it's all gonna play out? I don't know. But I'm very glad to be alive to watch this happen.
Anthony 29:00
All right, so we're coming up to the end. Dave, take us home. What have you learned about being human from listening to this series?
Dave 29:07
Soon, we'll see AI creating AI. So Alex mentioned, you know, it's beautiful that right now humans have the ability to create AI. I think we're not not that far off from seeing AI start to create AI, to having billions of AI workers creating billions of AI workers. And that becomes very interesting. I think that creating value for users doesn't mean replicating human behavior. That's one thing that I've heard sort of consistently throughout this, that replicating human behavior, in some ways is really interesting. But you know, you talked about the paradox that this is a hard thing to do. But we don't need to replicate human behavior in order to create value for users, and that we're not monolithic loved that - we're a bundle of different behaviors and maybe AI will start to peel off the things that we don't like to do: the email and the painful interactions that we prefer not to do and allow us to focus on the stuff that we love to do. So I think our lives would be fuller in the future as a result of AI.
And then, you know, Anthony, you made a comment in one of the episodes about wishing that there was evolutionary pressure to optimize for math nerds. I invite you to come out and hang out in Silicon Valley. That is exactly what Silicon Valley is, like all the time, and so come hang out. But I think the next chapter will democratize the creation of technology. So I think that with AI folks that don't have computer science degrees, folks that didn't, you know, geek out on video games like we did in in our early lives and put computers together in our basements, like folks that are experiencing everyday problems throughout the entire country, will be able to create tools that will create value for everyone. That is very exciting, and I'm excited to be a part of that.
Anthony 30:43
I think that's a great place to finish.
So big thanks to Dave Munichiello for joining us this week.
This is the last episode for this series. Please listen to all the episodes which are available at your usual podcast platform. This is a GV podcast in a Blanchard house production. Our science producer was Hilary Guite, executive producer Duncan Barber with music by DALO.
I'm Anthony Philippakis.
Alex 31:10
I'm Alex Wiltschko.
Anthony 31:11
And this is Theory and Practice.